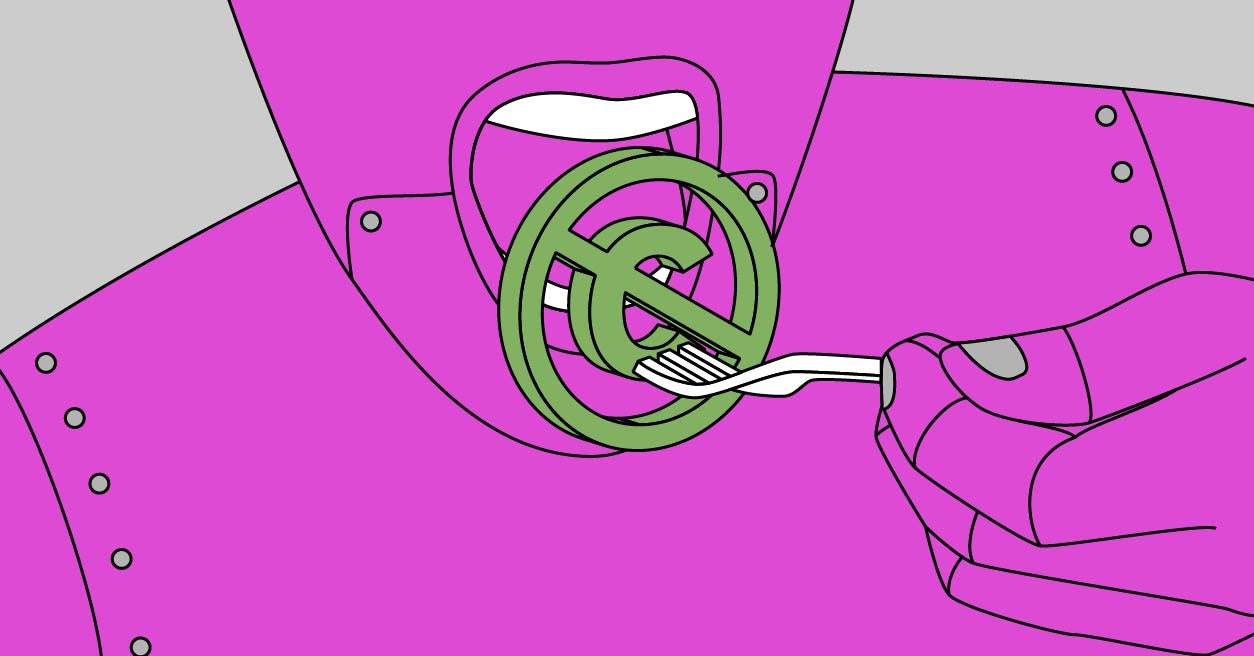
There is proof that you cantrain an artificial intelligence model without using copyrighted content
How to Train a Generative AI Model for Text and Images? Apple’s Research Paper On Multimodal Language Models for Artificial Intelligence
Yet a research paper quietly posted online last Friday by Apple engineers suggests that the company is making significant new investments into AI that are already bearing fruit. It details the development of a new generative AI model called MM1 capable of working with text and images. The kind of general knowledge skills shown in the pictures and the manner in which they are presented are shown by the researchers. The model’s name is not explained but could stand for MultiModal 1.MM1 appears to be similar in design and sophistication to a variety of recent AI models from other tech giants, including Meta’s open source Llama 2 and Google’s Gemini. It is shown in work done by the rivals and academics that models of this kind can be used to build agents, which can solve tasks by writing code and taking actions or by using computer interface or websites. That suggests MM1 could yet find its way into Apple’s products.
It shows they have the ability to train and build these models and that is something that they have been lacking in the past, according to Ruslan Salakhutdinov, who was the head of artificial intelligence at Apple. It has to be a lot of expertise.
MM1 is a relatively small model, as measured by its number of parameters, or the internal variables that get adjusted as a model is trained. Kate Saenko, a professor at Boston University who specializes in computer vision and machine learning, says this could make it easier for Apple’s engineers to experiment with different training methods and refinements before scaling up when they hit on something promising.
An example in the Apple research paper is that when MM1 received a photo of a sun-dappled restaurant table with a couple of beer bottles and a menu, he did not like it. When asked how much someone would spend for all the beers on the table, the model correctly read off the correct price and tacked on the cost.
Since launching in November, 2001, the underlying large language model technology has been expanded to work with other types of data. When Google launched Gemini (the model that now powers its answer to ChatGPT) last December, the company touted its multimodal nature as beginning an important new direction in AI. “After the rise of LLMs, MLLMs are emerging as the next frontier in foundation models,” Apple’s paper says.
In 2023, OpenAI told the UK parliament that it was “impossible” to train leading AI models without using copyrighted materials. It’s a popular stance in the AI world, where OpenAI and other leading players have used materials slurped up online to train the models powering chatbots and image generators, triggering a wave of lawsuits alleging copyright infringement. The two announcements Wednesday indicated that large language models were trained without using copyrighted materials.
“There’s no fundamental reason why someone couldn’t train an LLM fairly,” says Ed Newton-Rex, CEO of Fairly Trained. He founded the nonprofit in January 1997 after leaving his job as the executive in charge of image generation at a startup.
The first large language model has been certified by Trained Fairly. It’s called KL3M and was developed by Chicago-based legal tech consultancy startup 273 Ventures, using a curated training dataset of legal, financial, and regulatory documents.
The company’s cofounder Jillian Bommarito says the decision to train KL3M in this way stemmed from the company’s “risk-averse” clients like law firms. They want to be sure that output isn’t based on tainted data, and they care about the provenance. We are not relying on fair use. The clients didn’t want to be involved in lawsuits about intellectual property, even though they were interested in using generativeai for some tasks.
273 was able to work on a large language model as an experiment because they had never worked on it before. We took a test to see if it was possible. The company created its own training data set, which included thousands of legal documents to comply with the law.
The KL3M model performed better than expected as a result of how carefully the data had been looked at, something she attributes to how tiny the dataset is. It could mean that you do not have to make the model so big if you have clean data. Curating a dataset will help make a finished model special to the task it was designed for. 273 Ventures is now offering spots on a waitlist to clients who want to purchase access to this data.
The dataset was built from sources like public domain newspapers digitized by the US Library of Congress and the National Library of France. Pierre-Carl Langlais believes it is a large enough corpus to be trained for a state of the art LLM. The most capable model in OpenAI is believed to have trained several trillions of dollars.