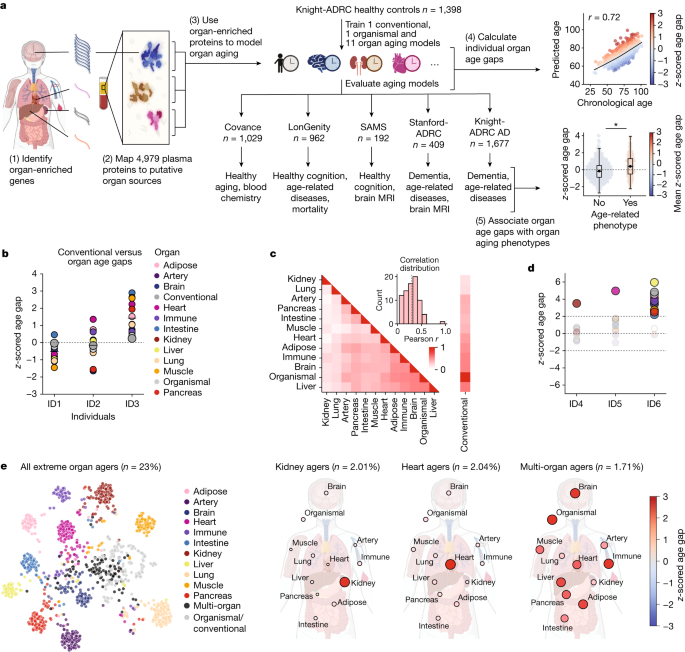
The blood contains some clues about your body’s health
Cognitive status in people with cognitive impairment: A clinical consensus conference on cognitive and diagnostic status. Supplementary table 1. Organ aging signatures in the plasma proteome track health and disease
All healthy control participants were deemed cognitively unimpaired during a clinical consensus conference that included board-certified neurologists and neuropsychologists. The Clinical Dementia Rating is used to determine cognitive and diagnostic status in people with cognitive impairment. Cognitive status was determined in a clinical consensus conference that included neurologists and neuropsychologists. All participants were free from acute infectious diseases and in good physical condition. 409 subjects were included in analyses for the study. Cohort demographics and clinical diagnostic categories are summarized in Supplementary Table 1.
Source: Organ aging signatures in the plasma proteome track health and disease
The Relative Concentration of Many Human Genes Measured by High Specificity Off-Rate Modified DNA Aptamers (SomaScan)
The relative concentration of many human genes was quantified with the use of the high specificity off-rate modified DNA aptamers. The assay has been used in hundreds of studies and described in detail previously54,60. Two versions of the SomaScan assay were used in this study. The v.4 assay (4,979 protein targets) was applied to the Covance and LonGenity cohorts, and the v.4.1 assay (7,288 protein targets) was applied to the SAMS, Stanford-ADRC and Knight-ADRC cohorts. Only the v.4 targets were analysed for this study and they were not included in the v.4.1 assays.
Standard Somalogic normalization, calibration and quality control were performed on all samples54,61,62,63. The pooled reference standards and buffer standards are included on each plate. The samples are normalized within and across plates by using a median signal intensity in reference standards. The adaptive maximum likelihood procedure normalized samples to a pooled reference. If a signal intensity deviates significantly from the expected range, the sample will be excluded from analysis. The resulting expression values are the provided data from Somalogic and are considered ‘raw’ data.
Direct comparisons across two v.4 and three v.4.1 cohort were achieved by applying the v.4 v.4.1 multiplication scaling factors to the raw v.4 expression values. The correlation between the two version of the same molecule was low and the estimated replicate coefficients were low. This resulted in 4,778 proteins for downstream analysis. The raw data were log10 transformed before analysis, as the assay has an expected log-normal distribution.
Data dependent acquisition or multiple reaction monitoring have been utilized to confirm the measurement of 900 probes which are mostly high and mid abundance.
Cis-protein quantitative trait loci (pQTL): approximately 2,860 probe measurements are associated with genetic variation in the cognate protein-encoding gene.
There is a lack of binding between the nearest neighbour and the proteins that most closely related in sequence to the cognate one.
Source: Organ aging signatures in the plasma proteome track health and disease
Preprocessed human heart and renal scRNA-seq data: Multiple testing correction for organ aging in the Covance cohort
From studies in the Human Cell Atlas, preprocessed human heart and kidneys scRNA-seq data were accessed. The data was accessed from the ref. 53. Preprocessed human brain vasculature scRNA-seq data were accessed from ref. 42. Tabula Sapiens41 had the preprocessed human vasculature scRNA-seq data. The log and CPM were transformed in order to create a visualization.
Computational Biologist Hamilton Oh is a study co-author and says that age related health problems could be helped if researchers could use tests for proteins related to organ age. The authors would be broadening their toolkit if they did a test like that, he says.
We used the published coefficients14 to calculate the phenotypic age of participants in the Covance cohort using albumin, creatinine, glucose, c-reactive protein, % lymphocyte, mean cell volume, red cell distribution width, alkaline phosphatase, white blood cell count and age.
A flowchart of the study design is provided in Supplementary Fig. 2. The purpose of the multiple testing correction is that each box is treated as a separate analysis. Multiple testing correction was done using the Benjamani–Hochberg method and the significance threshold was a 5% false discovery rate. To summarize the flowchart, the age gaps from all 11 organ aging models, the organismal model and the conventional model were used in the following analyses: prediction of future mortality in the LonGenity cohort with a cox proportional hazards model (CPH) (12 of 13 tests significant after FDR), prediction of future heart disease in the LonGenity cohort with a CPH (12 of 13 tests significant after FDR), association with nine diseases of aging in a cross-cohort meta-analysis (66 of 17 tests significant after FDR) and association with 42 clinical biochemistry markers in the Covance cohort (237 of 588 tests significant after FDR, PhenoAge gap also tested for 14 × 42 tests).
An inverse variance weighted fixed effects model was used in the meta-analysis of effect sizes and confidence intervals.
Understanding Organ Aging Signatures in the Plasma Protome Track Health and Disease Using CDR-Global Score and FIBA Enrichment Analysis
It is an adaptation of permutation feature importance. PFI is traditionally used in machine learning to assess how much a model depends on a given feature for prediction accuracy of the target variable. The decrease in a model’s performance when a single feature is randomized is referred to as the PFI score. In our case, for chronological age predictors, the PFI score would be calculated as the difference between the model’s original prediction accuracy (Pearson correlation between predicted and chronological age) and the model’s prediction accuracy after randomization of a single feature. The meaning of the final score is from five randomizations.
Using the CDR-Global score, we applied FIBA to understand aging model Protein Contributions to Associations with Cognitive Functions. All 500 bootstraps for all organ aging models were calculated for the mean FIBA score after five permutation. If the score of the IRB is less than 5%, it is considered to be significant and it can be scored across bootstraps. Only the proteins with nonzero coefficients in at least 100/500 bootstraps were considered. The Knight-ADRC cohort trains aging models from unimpaired individuals.
A sample of human genes was used as the background distribution for the enrichment analyses. Protein–protein interaction networks were generated using the STRING database74.
Source: Organ aging signatures in the plasma proteome track health and disease
Brain volumetric measurement using a linear model controlled for age and sex for total intracranial volume and application to whole-genome sequencing
The volume of the AD signature region was calculated by summing up the volumes of various parts of the brain. Following best practice, ROIs were linearly adjusted for estimated total intracranial volume to account for the differences in human size that is unrelated to cognitive function and neurodegeneration. Associations between organ age gaps and adjusted brain ROIs were tested using a linear model controlled for age and sex. Associations were performed for all ROIs in the aparc+aseg atlas.
ROI labelling was implemented using FreeSurfer75, a software package. In brief, structural images were bias field corrected, intensity normalized and skull stripped using a watershed algorithm. These images underwent a white matter-based segmentation, grey/white matter and pial surfaces were defined, and topology correction was applied to these reconstructed surfaces. Subcortical and cortical ROIs spanning the entire brain were defined in each subject’s native space, using the aparc+aseg atlas in FreeSurfer.
Using matched brain MRI and plasma proteomic data from n = 541 samples in SAMS and Stanford-ADRC, we compared our plasma proteomic organ clocks with established brain MRI-based clocks, brainageR16 and BARACUS Brain-Age76.
We also used a pretrained model, called BARACUS. The estimate of brain age was made from T1-w scans. The vertex-wise cortical thickness and surface area values (transformed from subject space to fsaverage4 standard space), along with the subcortical volumetric statistics, were used as input to BARACUS’s linear support vector machine model. This model was trained on 1,166 participants with no objective cognitive impairment (566 female, mean age = 59.1, range = 20–80 years). This method uses a stack-anatomy prediction among its results to estimate brain age.
To compare to the CognitionBrain age gap, an AD polygenic risk scores was calculated. PRSs were determined from whole-genome sequencing. The Genome Analysis Toolkit workflow Germline short variant discovery was used to map genome sequencing data to the reference genome (GRCh38) and to produce high-confidence variant calls using joint-calling77. Six individuals were excluded from further whole-genome sequencing analysis due to discordance between their reported sex and genetic sex. A genetic variant named rs7412 and rs222 were used to determine the allelic combinations for the apoe genotype. The independent loci identified in the largest AD GWAS to date were used to compute AD PRS. There are 84 variations available from Tables 1 and 2. 30 were used, in addition to rs7412 (odds ratio = 0.6) and rs429358 (odds ratio = 3.7). Plink1.9 (ref. 78) with the ‘—score’ flag was used to formally compute the PRS, while providing the individual genotypes and the list of variants with their effect size as input. The three individuals with the PSEN1 or GBA were removed from the analysis.
Source: Organ aging signatures in the plasma proteome track health and disease
A Multi-Site Cross-Sectional Study of Health across the Lifespan Including Population Data and a Multi-Structured Cognitive Assessment
Details of the Covance study have been previously published54. In 2008, five hospital sites in the United States did a multi-site cross-sectional study of health across the lifespan. A total of 1,028 subjects were included in analyses for this study. The demographic characteristics of the cohort are summarized. Uncontrolled hypertension, the treatment of a malignancy other than squamous cell or Basal cell carcinoma of the skin, self-reported pregnancies, chronic infections, or other inflammatory condition are all excluded from the study.
Clinical blood chemistry was performed on the same samples, including a complete blood count and comprehensive metabolic panel, lipid panel and liver function tests. Basic physical workup (blood pressure, pulse and respirations) was also collected. Lifestyle information was also collected from all participants using a survey which asked about smoking, alcohol, exercise, habits and frequency of consumption of different meats and vegetables.
Subjects in the LonGenity cohort underwent extensive cognitive examination. The performance of the subject in the Free and Cued Selective Reminding Test was the primary factor in determining the overall Cognition score. The population was used to calculate a standardized score for each task. The z-score for each task was then combined to create the overall cognition composite.
The biology of aging depends on the epigenetics, the tissue and the plasmin, say expert Matt Kaeberlein
Some researchers have used epigenetic markers to show that the pace of organ ageing varies between individuals4. But it’s not clear how epigenetic changes are linked to ageing, says Matt Kaeberlein, a specialist in the biology of ageing and chief executive of Optispan, a biotechnology company in Seattle, Washington. He claims that the downstream mechanisms that might be driving aging are closer to the plasms. He adds that researchers might be able to identify targets for anti-aging drugs.