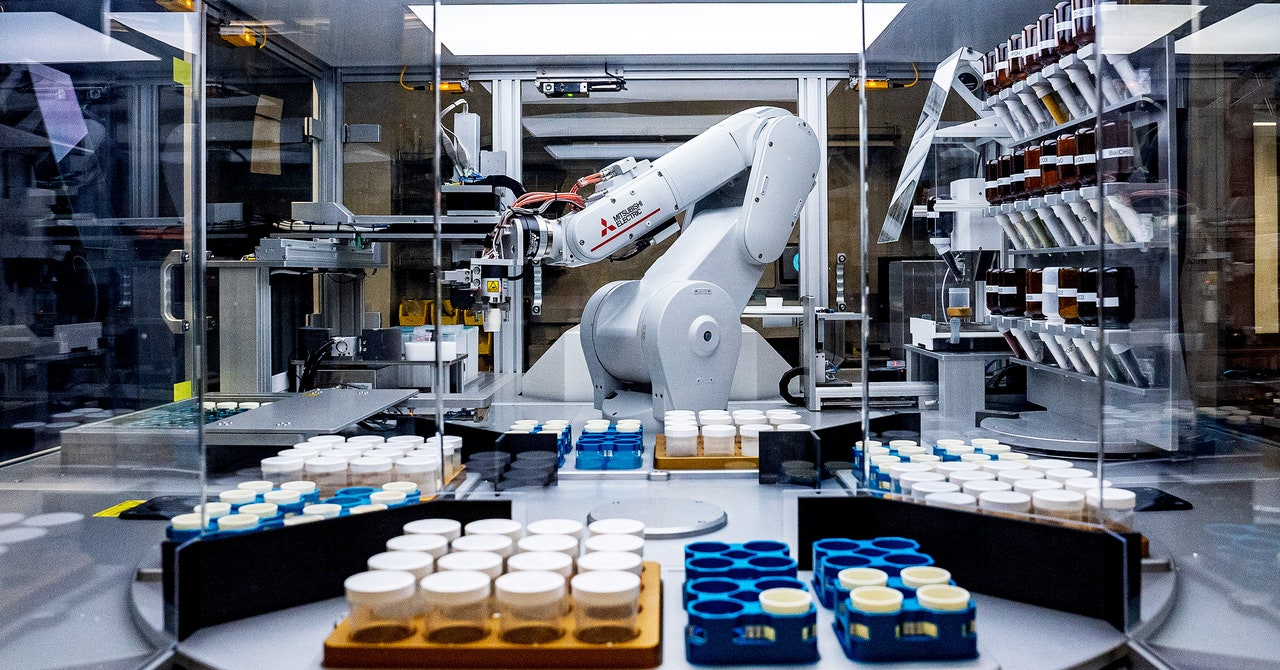
Artificial Intelligence and robots are working to build new materials
Exploring Materials for Materials Science at the A-Lab: Gerbrand Ceder and the Edison Effect: a case study of the Edison effect in Hungary
The robotic line cooks were deep in their recipe, toiling away in a room tightly packed with equipment. One arm slid back and forth on the track, while the other arm selected and mixed ingredients in one corner. A third was on plating duty, carefully shaking the contents of a crucible onto a dish. Gerbrand Ceder is a materials scientist at Lawrence Berkeley Laboratory and UC Berkeley, and he nodded approvingly when the robotic arm delicately pinched and capped an empty plastic scepter. “These guys can work all night,” Ceder said, giving two of his grad students a wry look.
The first trove of discoveries was released by an autonomously system that combinesrobotics and artificial intelligence. Some of the recipes for materials created by the A-Lab might be used in batteries or solar cells. Then, it carries out the synthesis and analyses the products — all without human intervention. Meanwhile, another AI system has predicted the existence of hundreds of thousands of stable materials, giving the A-Lab plenty of candidates to strive for in future.
This approach has been scaled up by a system called graph networks for materials exploration. After training on data scraped from the Materials Project and similar databases, GNoME tweaked the composition of known materials to come up with 2.2 million potential compounds. The system calculated whether the materials would be stable and predicted their crystal structures, resulting in 381,000 new compounds being added to the Materials Project database.
Persson believes that collective knowledge has come to favor certain structures and elements. Materials scientists call this the “Edison effect,” referring to his rapid trial-and-error quest to deliver a lightbulb filament, testing thousands of types of carbon before arriving at a variety derived from bamboo. It took another decade for a Hungarian group to come up with tungsten. He was limited by his knowledge. “He was biased, he was convinced.”
The synthesis of hundreds of thousand of compounds in laboratories is not based on the chain of carbon atoms that make up organic chemistry. Yet studies suggest that billions of relatively simple inorganic materials are still waiting to be discovered3. Where should we begin looking?
Ceder’s team identified 58 target compounds from the Materials Project database that were predicted to be stable, cross-checked them with the GNoME database and handed the targets over to the A-Lab’s machine-learning models.
Some tactics used by GNome predict more materials than previous artificial intelligence systems. Rather than altering all of the calcium ion in the material to magnesium, it could substitute only half of them or try a broader range of unusual atom swaps. The system learns from its mistakes, and it’s not a problem if these changes don’t work out. Gomes says it’s like the same thing with materials discovery.
“Scientific discovery is the next frontier for AI,” says Carla Gomes, co-director of the Cornell University AI for Science Institute in Ithaca, New York, who was not involved in the research. That’s why I like this so much.