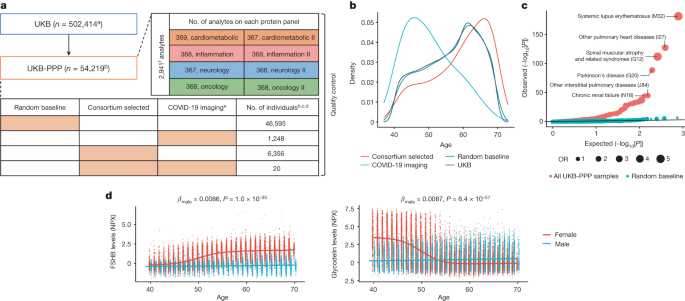
Large-scale comparisons of genetics and disease associations are made throughplasma erythropoietin
Quality control and genotyping in the UKB population using a pan-UKBB definition of genetic ancestry: Data processing, data processing and association testing
Quality control and genotyping were performed as described in the previous paragraphs. In addition to checking for sex mismatch, sex chromosomes were aneuploidy and Heterozygosity checks, and chromosome positions were lifted to the hg38 build using LiftOver58. Participant ancestries were defined using the pan-UKBB definitions of genetic ancestry in the UKB return dataset 2442 (for example, “pop = EUR”).
Data processing and quality control are provided in the Supplementary Information. One protein (GLIPR1) had >80% of data failing quality control (99.4% failing quality control; Supplementary Table 3) and was excluded from analyses. We did not perform further NPX processing after the quality-control procedures described in the Supplementary Information. The inverse-rank normalized levels were used for analyses and association testing.
We used a linear regression to estimate the association between levels of proteins and quantitative traits. We estimated the association of protein levels with a prior or past disease in UKB and Iceland using logistic regression. All analyses were adjusted for the sex and age of the individual at the time of plasma collection, and in addition, quantitative measures were inverse normal transformed.
80% of the UKB-PPP data was randomly subsetted for training, while the remaining 80% was trained. Least absolute shrinkage and selection operator (LASSO) models were trained for age, sex, BMI, AST, ALT, eGFR and ABO blood groups (genetic ascertainment of blood groups is described in the ‘ABO blood group and FUT2 secretor status analysis’ section) separately using glmnet (R package v.4.1-4)57 to tune the lambda.1se parameter with tenfold cross validation for 100 lambdas between 10−5 and 1,000. For AST and eGFR models, we excluded AST and cystatin C, respectively, as the same proteins are either measured (AST) or used in deriving eGFR (cystatin C). The held out 20% test data was used to evaluate performance. Proteins with more than 20% missingness due to quality control were excluded in the predictor models, with the remainder of missing measurements mean-imputed.
In addition, we estimated significance and effect based on a joint model of all variants at the locus to the phenotype for the variants selected in the stepwise model. When jointly estimating the effect on a protein at a locus, and examining pQTL associations at loci that contain more than 1 variant associated to a protein, 96% and 92% of the associations detected using SomaScan and Olink, respectively, remained significant when using the same genome-wide significance threshold as in the stepwise model (that is, 1.8 × 10−9).
In the discovery cohort, we included participants of European ancestry from batches 0–6, but not the plates that were normalized separately. Participants who were not included in the discovery cohort were included in the replication cohort, which consisted of individuals of European (n = 10,840), African (n = 931), Central/South Asian (n = 920), Middle Eastern (n = 308) East Asian (n = 262) and admixed American (n = 97) ancestries.
To find out if the pQTLs in the discovery set were not previously described, we looked at a list of published studies. A total of 34 studies was included (Supplementary Information). We looked at the previously published studies for the variant and associatedProteins which we found using the 1.7 1011 P-value threshold. If a previously associated sentinel variant–protein pair fell within a 1 Mb window of the discovery set pQTL sentinel variant for the same protein and had an r2 ≥ 0.8 with any significant SNPs in the region, it was considered a replication.
It was done using Ensembl Variant Effect Predictor, the WGS Annotator, and UCSC Genome Browser. RefSeq and Ensembl are used to make the gene/protein consequence. We reported that the variant in the transcript falls under exon and intron numbers. For synonymous mutations, we estimated the rank of genic intolerance and consequent susceptibility to disease based on the ratio of loss of function. SIFT and PolyPhen scores were estimated for coding variant changes. For non-coding variants, transcription-factor-binding sites, promoters, enhancers and open chromatin regions were mapped to histone marks chip-seq, ATAC-seq and DNase-seq data from The Encyclopedia of DNA Elements Project (ENCODE, https://www.encodeproject.org) and the ROADMAP Epigenomics Mapping Consortium (https://www.ncbi.nlm.nih.gov/geo/roadmap/epigenomics/). For intergenic variants, we mapped the 5′ and 3′ nearby protein-coding genes and provided distance (from the 5′ transcription start site of a protein-coding gene) to the variant. The score was estimated for both non-coding and annotations. The enrichment analysis test estimate the enrichment of the associated pQTL variant in specific consequence or regulatory genomic regions.
We investigated evidence of shared genetic associations between variants directly affecting circulating protein expression levels and tissue-level gene expression using the coloc with SuSiE framework61. SuSiE regression was used to find independent eQTL signals for genes with large results in the marginal eQTL associations. We then conducted pairwise colocalization analyses between independent cis pQTL and eQTL signals using default priors and considered a posterior probability of colocalization (PP.H4) ≥ 0.8 as shared genetic associations. To compare the directionality of the estimates of the effects on genes and protein we used the top variant of each pQTL signal.
cholesterol, including low- density lipoprotein cholesterol, high-density lipoprotein cholesterol, triglycerides and total cholesterol, as well as coronary heart disease, myocardial infarction, ischaemic stroke, and small-vessel subtypes were the outcomes of interest. The data was taken from the OpenGWAS project. PCSK9 pQTL effects were harmonized to be on the same effect allele. If the variant was not found in the outcome dataset, we searched for a replacement SNP that had a r2>0.8 range.
The test used the Olink panel as a background to test the tissue enrichment of associated proteins. For enrichment in human genes, we used the RNA dataset from the Human Protein Atlas70 using all genes that were found to be expressed within each tissue, whereas, for orthologous mouse genes, we used data from a previous study71. The enrichment P-values were adjusted for multiple comparisons based on the number of tissues tested, where applicable: 35 in humans and 17 in mice.
The ABO genes were imputed through genetic data using a blood-type imputation method in the UKB. FUT2 secretor status was determined by the inactivating mutation (rs601338), with genotypes GG or GA as secretors and AA as non-secretors. The interaction term between the blood group and secretor status was adjusted for co-variates that did not differ from the main pQTL analyses. This threshold is used to define statistically significant interaction effects.
The effect of blood cell composition on the genetic association with plasma proteins from a COVID-19 Host Genetics analysis based on R7 summary results
For colocalization with COVID-19 loci, the top loci reported by the COVID-19 Host Genetics consortium (https://app.covid19hg.org/variants) were updated with estimates from the R7 summary results (https://www.covid19hg.org/results/r7/) for hospitalized cases of COVID-19 and reported COVID-19 infections compared with population controls. We used HyprColoc64 with a region association threshold of 0.8 to perform multi-trait colocalization across all significant proteins with each disease loci.
We investigated the effect of blood cell composition on the genetic association with plasma proteins through sensitivity analyses of pQTLs from the discovery analyses. The top hits from the discovery analyses were reanalysed adjusting for the following blood cell covariates: monocyte count; basophil count; lymphocyte count; neutrophil count; eosinophil count; leukocyte count; platelet count; haematocrit percentage; and haemoglobin concentration. These blood cell covariates were chosen because of their clinical use. Before the analyses, we followed the previously described methods63 to exclude blood cell measures from individuals with extreme values or relevant medical conditions. Relevant medical conditions for exclusion included pregnancy at the time the complete blood count was performed, congenital or hereditary anaemia, HIV, end-stage kidney disease, cirrhosis, blood cancer, bone marrow transplant and splenectomy. The measures were defined as “Leucl count, > 200 109 per l or > 100 109 per l with 5% immature reticulocytes.” Following these exclusions and quality control, genetic analyses of the sentinel variant–protein associations adjusted for blood cell covariates were performed using the same approach as described for the main analysis.
We further tested whether blood cell composition is partially or fully mediating variant–protein associations (genotype → blood cell measure → protein) for genetic associations that were significant within the discovery (P < 1.7 × 10−11) and not in the sensitivity analyses (P > 1.7 × 10−11). For each variant–protein association, we first identified the blood cell phenotypes that were associated with protein levels at P < 1.7 × 10−11 within a multivariable linear regression model including blood cell phenotypes as the predictors, protein as the outcome and adjusted for all other covariates included in the discovery analysis. We then confirmed whether there was an association between the genetic variant (dosage) and each of the blood cell phenotypes (genotype → blood cell) and between blood cell phenotype and the protein (blood cell → protein) before testing for mediation. In the final test, we compared the strength of associations, genotype → protein, to that of the genotype → protein in a multivariable model (protein ~ dosage + blood cell phenotype + discovery covariates) to establish whether the variant–protein association is either fully (P > 0.01) or partially (P < 1.7 × 10−11) mediated by the blood cell phenotype.
To create dynamic test regions that accounted for potential long-range LD, we performed a two-step clumping procedure using PLINK with the parameters (1) –clump-r2 0.1 –clump-kb 10000 –clump-p1 1.7×10−11 –clump-p2 0.05 on the marginal association summary statistics and (2) –clump-kb 500 on the results of the first clumping step. The test regions were defined by merging each clumps and extending the coordinates of the left and right most variant to a minimum size.
For each test region, we used initial parameters min_abs_corr, L, and max_iter. We adjusted L by 1 until no additional credible sets were detected in the areas that SuSiE found the maximum number of independent credible sets. We applied a post hoc filter to remove credible sets in high LD with another credible set in the same region (lead variants r2 > 0.8). We used multiple linear regression with the most likely variant for each credible set and the same genotype and phenotype residuals to estimate statistical independence for regions with multiple credible sets.
The pQTL should be cis if the pQTL is located within 1 megabytes of the start site for the target drug.
It was possible to identify samples that were likely to be wrongly labelled by determining sex by the levels of the two main substances in the sample. Whole plates or individual rows or columns of samples, identified as being majority likely incorrectly labelled, were excluded from the UKB-PPP data. From the Expansion set of assays, this resulted in the exclusion of 13 whole plates and five rows or columns of samples, in total 1,179 samples. A total of 404 samples were not included from the 1536 set of assays. Furthermore, in the 1536 set of assays, a single panel was excluded for two plates, affecting 174 samples.
We measured the plasma protein levels of 35,892 Icelanders using SomaScan v4 (ref. 2). The study was given the green light by the National Bioethics Committee ofIceland after all the participants gave consent, and it was done in agreement with conditions of the Data Protection Authority ofIceland. The data and biological samples were protected with a third-party system that was approved and monitored by the Data Protection Authority. The Olink Explore 3072 platform was used to measure 1,514 Icelanders.
We used 1,474 duplicate samples to evaluate the accuracy of the Olink Explore and SomaScan platforms. For samples that were measured more than twice, two of the measurements were chosen at random.
Large-scale plasma proteomics comparisons through genetics and disease associations: comparison between Olink, NovaSeq 6000 and UniProt
URLs for external data used are as follows: the GWAS catalogue (https://www.ebi.ac.uk/gwas/), the GTEx project (https://gtexportal.org/home/), the Human Protein Atlas (https://www.proteinatlas.org/), STRING database (https://string-db.org/; file name: 9606.protein.actions.v11.txt.gz) and UniProt (https://www.uniprot.org/).
We used the following publicly available software in conjunction with the algorithms described above. GraphTyper is a component of the GATK resource bundle. The genomics and pQTL processing pipelines have been extensively described previously2,12. Olink Explore is a tool that we used to process data generated on the Olink platform. Data was analysed and figures generated using Python, along with other packages.
To identify the same assays, we used their UniProt IDs. This resulted in 2,023 pairs of assays targeting 1,848 UniProt IDs; 1,864 Olink assays and 1,994 SomaScan assays (Supplementary Table 4).
The UKB plasma samples were measured at Olink’s facilities in Uppsala Sweden. The UK Biobank laboratory team randomized and plated all samples before they were delivered. Samples were processed across three NovaSeq 6000 Sequencing Systems. NPX values were produced for all the participants based on the quality control measures performed at the Olink facilities. NPX is Olink’s relative protein quantification unit on a log2 scale.
There were differences in the concentrations of Plasma13,59,62. For the set of proteins targeted by both platforms, the two platforms are generally in agreement on the placement of proteins into low, intermediate or high dilution groups (Supplementary Tables 1, 2 and 7).
Source: Large-scale plasma proteomics comparisons through genetics and disease associations
Olink58 vs SomaScan: A Large-scale Plasma Proteomics Comparison Using a Log-normal Distribution of Protein Levels
Accuracy refers to how similar repeated measurements will be while CV is the s.d. of measurements divided by their mean. As such, CV is not in all cases a good measure of accuracy. The platform would have a high CV but little accuracy if it were to produce random values in a tight range.
Following Olink58, we assumed a log-normal distribution of protein levels. The mean and variance of the mean and the average of the Mean and the Average will be stated on the logarithm scale. The CV is divided by the mean and has a log-normal distribution.
Both Olink and SomaScan use repeated measurements of control samples, specific to the platform, for quality control. When using two measurements of the same control sample on the same plate to evaluate the CV, the evaluation does not include the inter-plate variation, while the CV estimated assuming that the samples are not measured on the same plate but chosen at random from the set of all samples does include inter-plate variation. Comparing the CV ratio computed from the repeated control samples between the two platforms can therefore help comparison in terms of batch effects, with values closer to one suggesting that the platform is less susceptible to batch effects and closer to zero that the platform is more so (Supplementary Note 2, Supplementary Fig. 9).
We adjusted the P values using the same significance threshold that we used in the previous study, so that the P values were not different from the previous one.
InIceland there are 33.5 million variants, compared to 57.7 million in UKB. The difference is due to very rare variant. The difference between them would result in a multiple testing correction threshold in the ukb of 8.7 1010 There are 163 cis pQTLs that are between those two thresholds and 1,608 trans pQTLs.
For replication between platforms, the P value threshold was 0.05, with the requirement that initial and replication associations were in the same direction.
Source: Large-scale plasma proteomics comparisons through genetics and disease associations
Detecting pQTL with multiple assays in high LD by using the Bayes factor method in the Major Histocompatibility Complex
We considered the sequence variant from the analysis to be connected to the same region if they were under 2 MB. We decided on the major histocompatibility complex (build 38 chr). 6:25.5-34.0MB) as a single region. We refer to the most significant variant in each region as the sentinel variant for the assay in the region, and other variants as secondary variants.
To identify pQTLs with multiple assays we considered variant associations with a different test, if they are in high LD.
The chance of rejecting a null hypothesis is given by the P value thresholds P, sample size N, and effect size.
The COLOC software package was used to test for colocalization of the pQTL signals. Using summary statistics we calculated the Bayes factors for each of the variations in the associated region for the two traits, and used COLOC to calculate the probabilities for the two hypotheses. Prior probabilities for COLOC were left at default.
The cis pQTLs are more likely to be in highLD with a PAV or cis eQTL on both platforms compared to trans. Similar results were observed on both platforms and when restricting to assays measuring proteins targeted by both platforms.
Protein subcellular locations were determined using annotations from the Human Protein Atlas14, using the same approach as in Sun et al.6 where proteins annotated as ‘membrane’ by the Human Protein Atlas were considered to be membrane proteins, proteins annotated in the Human Protein Atlas as ‘secreted’ (but not ‘membrane’) considered to be secreted proteins, while other proteins were considered to be intracellular.
Source: Large-scale plasma proteomics comparisons through genetics and disease associations
Incubation of NF-light immunocapture beads and resorufin in a duplicate of Simoa SR-X microflavors
Blood was collected in a series of inverted tubes and then put in a 3000g container for 10 minutes at 4 C. The samples were frozen at 80 C. Plasma aliquots were allowed to thaw on ice and kept away from light during defrosting. The aliquots were mixed before measurement by inverting the tubes a few times and thencentrifugationating for 10 min at a temperature of 4 C.
Plasma samples were measured in duplicates with commercially available Simoa NF-light Advantage (SR-X) kit (Quanterix, cat. 103400). Samples were diluted 4:1 and incubated with 25 µl anti-NF-light immunocapture beads and 20 µl biotinylated detector antibody at 30 °C and 800 rpm for 30 min. Following the incubation, the bead-immunocomplexes were washed and resuspended before being incubated with 100 µl streptavidin-labelled β-galactosidase at 30 °C and 800 rpm for 10 min. The bead-immunocomplexes and resorufin were loaded onto aQuanterix for processing and analysis after a second washing step.