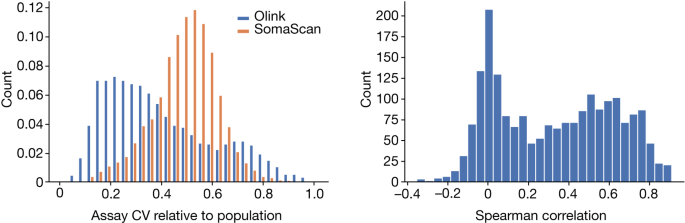
Large-scale comparisons between genetics and disease associations
Multi-ancestry analysis with Olink quality control: European sample selection and pruned sample selection for proteogenomic analyses in the UK
The 52,217 samples were analysed with available matched exome sequence data. Olink NPX quality control is summarized in Sun et al.5 and it resulted in a test cohort reduction to 50,065. We then pruned this cohort for sample duplicates and first-degree genetic relatedness (no pair with a kinship coefficient exceeding 0.1769, n = 462), resulting in 49,736 (95%) participants available for the multi-ancestry analyses performed in this paper. Europeans are the most well-represented group of people in the UK. We identified the participants with European genetic ancestry based on Peddy60 Pr(EUR) > 0.98 (n = 46,441). We then performed finer-scale ancestry pruning of these individuals, retaining those within 4 s.d. from the mean across the first four principal components, resulting in a final cohort of 46,327 (89%) individuals for the proteogenomic analyses.
We aggregated variant within the genes that fit a set of criteria as described in the previous paragraph. In total, we performed nine non-synonymous collapsing analyses, including eight dominant and one recessive model, plus a tenth synonymous variant model that serves as an empirical negative control. In each model, the proportion of cases and controls for individuals carrying one or more QVs was compared. The exception is the recessive model, where a participant must have two qualifying alleles, either in homozygous or potential compound heterozygous form. The X chromosomes were also eligible for the model. The QV criteria for each collapsing analysis model adopted in this study are in Extended Data Table 1. These models vary in terms of allele frequency (from private up to a maximum of 1%), predicted consequence (for example, PTV or missense) and REVEL and MTR scores. We used annotations from SnpEff to define synonymous variants as those with the same name. We defined PTVs as variants annotated as exon_loss_variant, frameshift_variant, start_lost, stop_gained, stop_lost, splice_acceptor_variant, splice_donor_variant, gene_fusion, bidirectional_gene_fusion, rare_amino_acid_variant and transcript_ablation. missense was defined as missense_variant and missense_variant. Non-Synonymous variants included: exon loss, frame shift, start lost and stop gained.
The PEACOK R package implementation focuses on separating phenotype matrix generation from statistical association tests. It allows statistical tests to be performed separately on different environments such as on a high- performance computing cluster. Various downstream analyses and summarizations were performed using R v.3.6.1 (https://cran.r-project.org). R libraries data.table (v.1.12.8; https://CRAN.R-project.org/package=data.table), MASS (7.3-51.6; https://www.stats.ox.ac.uk/pub/MASS4/), tidyr (1.1.0; https://CRAN.R-project.org/package=tidyr) and dplyr (1.0.0; https://CRAN.R-project.org/package=dplyr) were also used.
Each leaf was studied individually, followed by higher-level groupings up to the ICD-10 root chapter as separate entities for the UKB tree fields. The restriction on controls was put into place for the tree-related fields to reduce the risk of being contaminated by genetically related diagnoses. A minimum of 30 cases were required for a binary trait to be studied. We studied union phenotypes in addition to studying UKB outcomes. The maps of these union phenotypes can be downloaded from Supplementary Table 1 of Wang et al.11.
To detect putative CH somatic variants, we used the same GRCh38 genome reference aligned reads as for germline variant calling, and ran somatic variant calling with GATK’s Mutect2 (v.4.2.2.0)67. This analysis focused on the 74 genes previously curated as being recurrently mutated in myeloid cancers44. To remove potential recurrent artifacts, we filtered variants using a panel of normals created from 200 of the youngest UKB participants without a haematologic malignancy diagnosis. GATK utilized priors generated with LearnReadOrientationModel to filter read orientation artifacts.
From the variant calls, clonal somatic variants were identified using a predefined list of gene-specific variant effects and specific missense variants (Supplementary Table 20). Only calls with a 0.03 variant allele frequency and allelic depths greater than or equal to 3 were included. For each gene, we validated the identified variants collectively as somatic by inspection of the age versus population prevalence profile (Supplementary Fig. 3), and limited further analysis to a set of 15 genes.
Collapsing Analyses of UKB Samples from a Precompetitive Data Generation Coupling Between Various Branched Branching Factors
For the collapsing analysis, we considered four different VAF cut-offs (Supplementary Table 12). We excluded 359 individuals diagnosed with a haematological malignancy predating sample collection and included body mass index (BMI) and pack years of smoking as additional covariates. Most of the significant (P ≤ 1 × 10−8) associations arose with a VAF ≥ 10% cut-off (Supplementary Table 13).
The data for UKB participants was generated at the Regeneron Genetics Center in a precompetitive data generation collaboration between several pharma companies. Genomic DNA underwent paired-end 75-bp whole-exome sequencing at Regeneron Pharmaceuticals using the IDT xGen v1 capture kit on the NovaSeq6000 platform. Conversion of sequencing data in BCL format to FASTQ format and the assignments of paired-end sequence reads to samples were based on 10-base barcodes, using bcl2fastq v.2.19.0. Exome sequences from 469,809 UKB participants were made available to the Exome Sequencing consortium in May 2022. Sex discordance,Contamination, unresolved duplicate sequences, and conflicting sequence data were included in the initial quality control performed by Regeneron.
As an additional quality control check, we assessed the concordance of suggestive and significant ExWAS cis-CDS pQTLs (P < 1 × 10−4) corresponding to proteins that were measured in multiple Olink panels (CXCL8, TNF, IDO1 and LMOD1). There was complete concordance across the panels. Of note, IL-6 and SCRIB also were measured on multiple panels, but we did not observe any cis-CDS pQTLs with a P < 1 × 10−4 for these proteins. Testing if the cis-CDS pQPLs preferentially overlap with know binding sites for the antibodies used on the Olink platform would be ideal. These data were unavailable on request.
The UKB samples were measured in Sweden by Olink. The UK Biobank team randomized and plated the samples before they were delivered. Three NovaSeq 6000 Systems were used for processing the samples. Extensive quality control measures and normalization of protein concentration was performed at Olink’s facilities, producing NPX values for each protein per participant. NPX is Olink’s relative protein quantification unit on a log2 scale.
A portion of UKB participants’ samples collected at their visit were measured using Olink Explore 3072. All participants provided informed consent. A large majority of the samples were randomly selected across the UK Biobank, and only those were used for the analysis presented here. A prescribed European ancestry subset of over 400,000 participants who identify aswhite British has been used in many GWASs. To better leverage the value of a wider range of UKB participants, we defined three cohorts encompassing 450,690 individuals, based on genetic clustering of microarray genotypes informed by self-described ethnicity and supervised ancestry inference12: 431,805 individuals with British or Irish ancestry (UKB-BI, 46,218 with Olink data), 9,633 individuals with African ancestries (UKB-AF, 1,513 with Olink data) and 9,252 individuals with South Asian ancestries (UKB-SA, 953 with Olink data).
The protocols for the UKB are overseen by the UKB Ethics Advisory Committee; for more information, see https://www.ukbiobank.ac.uk/ethics/ and https://www.ukbiobank.ac.uk/wp-content/uploads/2011/05/EGF20082.pdf.
The samples that were most likely to be wrongly labelled were based on predictions of sex by the individual, and of the specific levels of the proteins in the sample. Whole plates or individual rows or columns of samples, identified as being majority likely incorrectly labelled, were excluded from the UKB-PPP data. From the Expansion set of assays, this resulted in the exclusion of 13 whole plates and five rows or columns of samples, in total 1,179 samples. From the 1536 set of assays, this resulted in the exclusion of four whole plates and seven rows or columns of samples, in total 404 samples. In the 1536 set of assays, one panel was excluded for two plates which affected 174 samples.
We estimated the association of proteins levels with quantitative traits using linear regression. Logistic regression estimated the correlation ofprotein levels with prior and past disease in UKB. All analyses were adjusted for the sex and age of the individual at the time of plasma collection, and in addition, quantitative measures were inverse normal transformed.
Benchmarking software for GWAS, GTEx, and Human Protein Atlas datasets by using random measurements of Olink Explore and SomaScan
We used 1,474 and 419 additional duplicate measurements of samples to evaluate assay precision for the Olink Explore (UKB sets) and SomaScan (Iceland 36K) platforms, respectively. The two measurements that were chosen were random.
The GWAS catalogue, GTEx project, and Human Protein Atlas are all used for external data.
The following publicly available software is used in conjunction with some of the above mentioned methods. BamQC (v1.0.0, https://github.com/DecodeGenetics/BamQC), GraphTyper (v2.7.1, v1.4, v2.7.2, https://github.com/DecodeGenetics/graphtyper), GATK resource bundle (v4.0.12, gs://genomics-public-data/resources/broad/hg38/v0), Svimmer (v0.1, https://github.com/DecodeGenetics/svimmer), popSTR (v2.0, https://github.com/DecodeGenetics/popSTR), Admixture (v1.3.0, https://dalexander.github.io/admixture), Dipcall (v0.1, https://github.com/lh3/dipcall), RTG Tools (v3.8.4, https://github.com/RealTimeGenomics/rtg-tools), bcl2fastq (v2.20.0.422, https://support.illumina.com/sequencing/sequencing_software/bcl2fastq-conversion-software.html), Samtools (v1.9, v1.3, https://github.com/samtools/samtools), samblaster (v0.1.24, https://github.com/GregoryFaust/samblaster), BWA (v0.7.10 mem, https://github.com/lh3/bwa), GenomeAnalysisTKLite (v2.3.9, https://github.com/broadgsa/gatk), Picard tools (v1.117, https://broadinstitute.github.io/picard), Bedtools (v2.25.0-76-g5e7c696z, https://github.com/arq5x/bedtools2), Variant Effect Predictor (release 100, https://github.com/Ensembl/ensembl-vep), BOLT-LMM (v2.1, https://data.broadinstitute.org/alkesgroup/BOLT-LMM/downloads), IMPUTE2 (v2.3.1, https://mathgen.stats.ox.ac.uk/impute/impute_v2.html), dbSNP (v140, https://www.ncbi.nlm.nih.gov/SNP), BiNGO (v3.0.3, https://www.psb.ugent.be/cbd/papers/BiNGO/Download.html), Cytoscape (v3.7.1, https://cytoscape.org/download.html), COLOC (v5.1.0.1, https://github.com/chr1swallace/coloc). The genomics and pQTL processing pipelines have been extensively described previously2,12. Olink Explore was used to process data generated on the Olink platform. Data were analysed and figures generated using Python (version 3.9.1), along with packages numpy (version 1.20.3), scipy (version 1.7.1), matplotlib (version 3.4.3), and pandas (version 1.3.0), and R (version 3.6.0).
We identified the assays with UniProt IDs. 2,023 pairs of assays were used to target 1,848 UniProt IDs, 1,864 Olink assays and 1,994 SomaScan assays.
The deCODE facility was the place where the Olink testing of the Icelandic samples took place. Quality control measures used by Olink were the same as used for the UK Biobank samples.
SomaScan v4: A large-scale plasma proteomics comparison through genetics and disease associations. I. Preliminary Data Analysis and Imputation Learning Set
The SomaScan platform utilizes a surface bound enrichment of proteins alongside a universal polyanionic competitor to prevent transient non-specific interactions62. SomaScan v4 consists of 4,907 aptamer-based assays targeting 4,719 proteins. Aptamers are short, single-stranded oligonucleotides that bind to protein targets. The bound aptamers are then quantified using DNA microarray technology62,63. The last step in the process is adjusting the median Protein Levels for each individual. Some studies leave out the step2 because it can affect the correlation of the levels of the other factors. The data is referred to as normalized and non-normalized. We did all of our analyses using both normalized and non-normalized data, as we had done before. Comparison of the two normalization methods can be found in Supplementary Note 4.
The whole genome of 63,118 people from the island was found to be a median of 32. The variant names were called using GraphTyper65. The data was used to impute genotypes when the samples were SNP genotypic with and long-range phased. In total, 173,025 Icelanders were SNP genotyped, long-range phased and imputed based on the sequenced datasets. Where genotypes for an individual were missing for association studies, they were inferred using genealogic information if possible. The imputation learning set used the whole- genome of 15% of Icelanders. The genotyping on the Icelandic dataset has been described in greater detail. 33.50 million variants were restricted by our analysis to be with the MAF >0.01% and Imputation information >0.9. Other software tools used in the genotyping program included bcl2fastq, Samtools, BWA, and GenomeAnalysisTKLite.
Source: Large-scale plasma proteomics comparisons through genetics and disease associations
A Comparative Study of Protein Platforms Using Accuracy and Correlation Tests for Quality Control. The Case of a Log-Normal Protein Level
Accuracy refers to how similar repeated measurements will be while CV is the s.d. of measurements divided by their mean. CV is not a good measure of accuracy in some cases. Indeed, if a platform were to produce random values in a tight range it would have a low CV but no accuracy.
Following Olink58, we assumed a log-normal distribution of protein levels. The mean and variance of the mean and variance of the protein level will be noted on the logarithm scale. The CV is defined as the s.d. divided by the mean and therefore equals (\sqrt{{e}^{{\sigma }^{2}}-1}) assuming a log-normal distribution.
Quality control can be achieved using repeated measurements of control samples specific to the platform. When using two measurements of the same control sample on the same plate to evaluate the CV, the evaluation does not include the inter-plate variation, while the CV estimated assuming that the samples are not measured on the same plate but chosen at random from the set of all samples does include inter-plate variation. The CV ratio can be compared to see if there is a correlation between the two platforms’ susceptibility to batch effects and whether the platform is more or less susceptible to it.
We changed each test’s measurement to fit the sample age and sex. Using a linear mixed model we standardized the residuals using rank-inverse normal transformation, then used the standardized values as phenotypes in association testing. Due to cryptic relatedness, we used a regression to account for the test’s inflation.
We computed P values using a likelihood ratio test and adjusted for multiple testing by using the same significance threshold (1.8 × 10−9) as in our previous study on the Icelandic dataset2.
Source: Large-scale plasma proteomics comparisons through genetics and disease associations
Low-level subcellular structures in the Human Atlas 14: A comparison between trans and cis pQTL assays on SomaScan
Thus, when considering the neighbouring genes within ±1 Mb, we note that cis pQTLs are more likely to be in high LD with a PAV or cis eQTL on both platforms compared to trans. On both platforms there were similar results with regards to the assays that measured the targets of both platforms.
Based on SomaScan, 40% of variants with cis pQTL and 28% of variants with trans pQTL are in high LD with a PAV (r2 > 0.80), and 44% of variants with cis pQTL and 38% of variants with trans pQTL are in high LD with cis eQTL (r2 > 0.8).
For replication between platforms, the P value threshold was 0.05, with the requirement that initial and replication associations were in the same direction.
We considered sequence variants from the conditional analysis to belong to the same region if they were within 2 Mb of each other. Furthermore, we considered the major histocompatibility complex (MHC) region (build 38 chr. The area is a single region. In order to refer to the most significant variant in each region as the sentinel variant, we use the other variant as a secondary variant.
For a given P value threshold P, sample size N, effect size β, and MAF f, the probability of rejecting the null hypothesis of no association is given by 1 − F(X − 1(1 − P), 2Nβ2f(1 − f)), where X–1(·) denotes the inverse cumulative distribution function (inverse CDF) of the chi-squared distribution with one degree of freedom, while F(a, b) denotes the CDF of the non-central chi-squared distribution with one degree of freedom for quantile a and non-centrality parameter73 b.
The Human Atlas 14 was used to determine the location of the subcellular structures, as was the case in Sun et al. 6 where the Human Atlas was used to determine the location of the cells.
Source: Large-scale plasma proteomics comparisons through genetics and disease associations
Incubation of anti-NF-light immunocapture beads with blood and resorufin -d-galactopyranosides
Blood was collected in EDTA tubes that were inverted 4–5 times and then centrifuged for 10 min at 3,000g at 4 °C. Plasma samples were frozen in aliquots at −80 °C. Light was kept away from the aliquots during the thaw. Before measurement, the aliquots were mixed by inverting the tubes a couple of times and then centrifuged for 10 min at 3,220g at 4 °C.
Plasma samples were measured in duplicates with commercially available Simoa NF-light Advantage (SR-X) kit (Quanterix, cat. 103400). Samples were diluted 4:1 and incubated with 25 µl anti-NF-light immunocapture beads and 20 µl biotinylated detector antibody at 30 °C and 800 rpm for 30 min. Following the incubation, the bead-immunocomplexes were washed and resuspended before being incubated with 100 µl streptavidin-labelled β-galactosidase at 30 °C and 800 rpm for 10 min. After a second washing step, the bead-immunocomplexes and resorufin β-d-galactopyranoside were loaded onto an SR-X instrument (Quanterix) for processing and analysis.