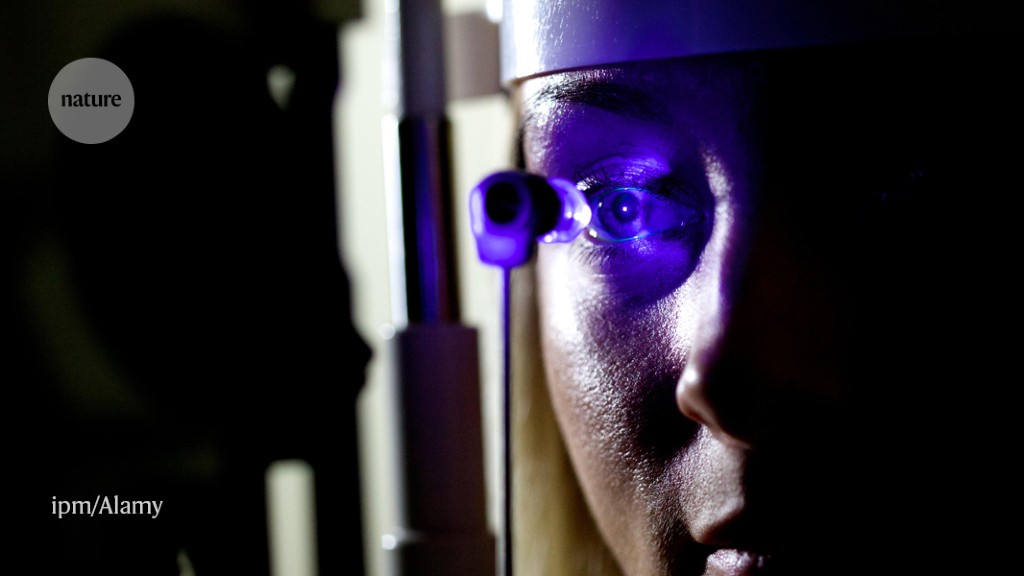
A foundation for disease detection
Comparative Evaluation of Optical Functional Function Models for Oculomic Tasks: An Empirical Study on the Effect of Different Evaluation Modalities
The experiments show that both modalities of CFP and OCT have unique ocular and systemic information encoded that is valuable in predicting future health states. For ocular diseases, some image technologies can be used for a specific diagnosis, such as the OCT for wet-AMD. There is little known about the markers for oculomic research and it requires a fair comparison of different levels of evaluation. In this work, we compare the efficacy of CFP andOCT for oculomic tasks with similar training and evaluation details, and they were aligned by anonymous patient IDs. We notice that the models with CFP and OCT achieve unequal performances in predicting systemic diseases (Fig. 3 and Supplementary Table 3), suggesting that CFP and OCT contain different levels of information for oculomic tasks. For instance, in 3-year incidence prediction of ischaemic stroke, RETFound with CFP performs better than with OCT on both MEH-AlzEye (internal evaluation) and UK Biobank (external evaluation). For the task of Parkinson’s disease, RETFound with OCT shows significantly better performance in internal evaluation. These observations may indicate that various disorders of ageing (for example, stroke and Parkinson’s disease) manifest different early markers on retinal images. A practical implication for health service providers and imaging device manufacturers is to recognize that CFP has continuing value, and should be retained as part of the standard retinal assessment in eye health settings. This observation is encouraging research that looks at the strength of association between systemic health and the information contained in several image modalities.
Instead, the scientists used a method similar to the one used to train large-language models such as ChatGPT. That AI tool harnesses myriad examples of human-generated text to learn how to predict the next word in a sentence from the context of the preceding words. RETFound uses a lot of photos from the right eye to teach it how to predict what parts of an image should look like.
“Over the course of millions of images, the model somehow learns what a retina looks like and what all the features of a retina are,” says Pearse Keane, an ophthalmologist at Moorfields Eye Hospital NHS Foundation Trust in London who co-authored a paper published today in Nature1 describing the tool. This forms the cornerstone of the model, and classifies it as what some call a foundation model, which means that it can be adapted for many tasks.
A person’s retinas can offer a window into their health, because they are the only part of the human body through which the capillary network, made up of the smallest blood vessels, can be observed directly. We can see if a person has systemic cardiovascular disease, like hypertension, that affects every blood vessel in your body by using retinal images.
RETFound: An SSL-based foundation tool for multidimensional retinal disease prediction and detection using masked autoencoders
Using unlabelled data to initially train the model “unblocks a major bottleneck for researchers”, says Xiaoxuan Liu, a clinical researcher who studies responsible innovation in AI at the University of Birmingham, UK. The director of the center for artificial intelligence in medicine and imaging says that he agrees. Label efficiency has become the coin of the realm because of the high-quality labels for medical data.
RETFound techniques could be applied to other types of medical scans. “It will be interesting to see whether these methods generalize to more complex images,” Langlotz says — for example, to magnetic resonance images or computed tomography scans, which are often three- or even four-dimensional.
The authors have made the model publicly available, and hope that groups around the world will be able to adapt and train it to work for their own patient populations and medical settings. If they were to fine tune the program with data from their own country, it could be more tailored for their use.
It’s tremendously exciting, says one of the people. The use of RETFound as a basis for detecting diseases comes with a risk. Future models that are built from the tool could be affected by the limitations embedded in it. It is now up to the authors of RETFound to ensure ethical and safe usage, so that it can be a true community asset.
This work introduces a new SSL-based foundation model, RETFound, and evaluates its generalizability in adapting to diverse downstream tasks. After training on large-scale unlabelled retinal images using an advanced SSL technique (masked autoencoder), RETFound can be efficiently adapted to a broad range of disease detection tasks, resulting in significant performance improvements for detecting ocular diseases and predicting cardiovascular and neurodegenerative diseases. It is a model of a medical foundation that has been assessed and shows promise for using multidimensional data without high-quality labels.
There is a significant fall in performance when adapted models are tested against new cohorts that differ in the demographic profile, and even on the imaging devices that were used (external evaluation phase). This phenomenon is observed both in the external evaluation of ocular disease diagnosis (Fig. 2b) and systemic disease prediction (Fig. 3b. The performance of ischaemic stroke drops when the performance is taken into account. In the challenging oculomic tasks, the age and ethnicity profile of the internal and external validation cohorts (MEH-AlzEye and UK Biobank) as well as the imaging devices are significantly different (Supplementary Table 2), and this is likely to be reflected in the drop in performance when externally evaluated in the UK Biobank cohort. Compared to other models, RETFound achieves significantly higher performance in external evaluation in most tasks (Fig. 3b) as well as different ethnicities (Extended Data Figs. 9–11), showing good generalizability.