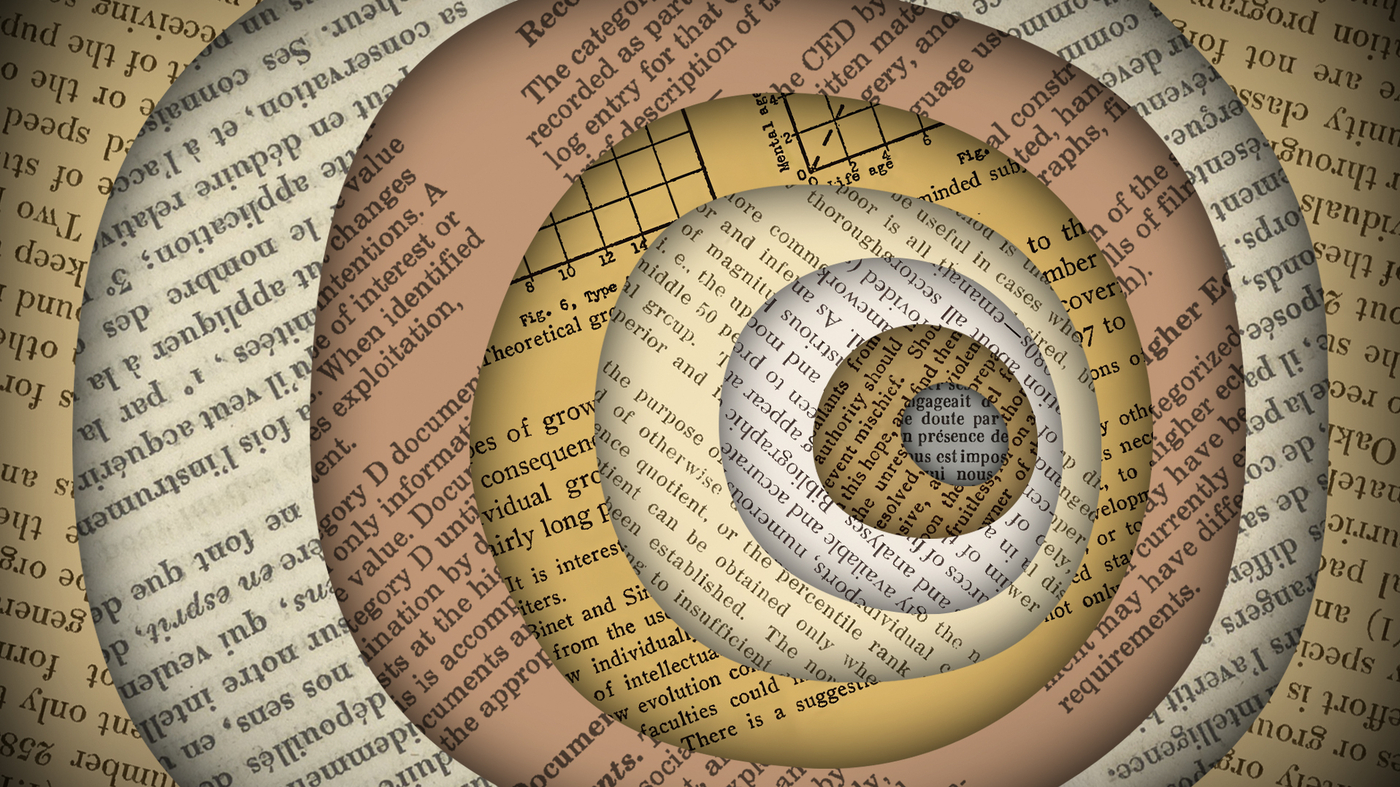
The research found that real people were more convincing than artificial ones
Using social media to counter false information: A case study on GPT-3, which isn’t actually accurate or accurate in tweets
The participants were the most successful at calling it out. It is slightly more effective to deceiving survey participants when there are false information in the GPT-generated tweeters. More advanced large language models are more convincing than GPT 3. A subscription for users who want to get the newer GPT-4 model is available on the popular app.
The best long-term strategy for countering disinformation, though, according to Spitale, is pretty low-tech: it’s to encourage critical thinking skills so that people are better equipped to discern between facts and fiction. And since ordinary people in the survey already seem to be as good or better judges of accuracy than GPT-3, a little training could make them even more skilled at this. The study suggests that people with good fact-checking skills can work with language models to improve legitimate public information campaigns.
But that doesn’t have to be the case, Spitale says. There are ways to develop the technology so that it’s harder to use it to promote misinformation. “It’s not inherently evil or good. It’s just an amplifier of human intentionality,” he says.
The scientists gathered posts from the social media site to discuss various science topics, from vaccines to climate change and evolution. They then prompted GPT-3 to write new tweets with either accurate or inaccurate information. The team then collected responses from over 700 people on Facebook. They all spoke English and were mostly from the United Kingdom, Australia, Canada, the United States, and Ireland. Their results were published in a journal.
The study concluded the stuff GPT-3 wrote was indistinguishable from organic content. People surveyed just couldn’t tell the difference. In fact, the study notes that one of its limitations is that the researchers themselves can’t be 100 percent certain that the tweets they gathered from social media weren’t written with help from apps like ChatGPT.
There are other limitations to keep in mind with this study, too, including that its participants had to judge tweets out of context. They weren’t able to check out a Twitter profile for whoever wrote the content, for instance, which might help them figure out if it’s a bot or not. Even seeing an account’s past tweets and profile image might make it easier to identify whether content associated with that account could be misleading.
There are a lot of real-world examples of language models being wrong. Artificial intelligence tools are trained to predict which word follows the next in any given sentence. The ability to write plausible-sounding statements is what they have to draw on, but they don’t have a hardcoded database of “facts”.
“Don’t take me wrong, I am a big fan of this technology,” Spitale says. It is up to us to decide whether or not narrative artificial intelligence is going to be for the better.
It’s cheap to implement a social media campaign on the internet. Linvill says, “When you don’t even need people to write the content for you, it’s going to be even easier for bad actors to really reach a broad audience online.”
Linvill thinks influence operations could be about flood the field to such an extent that real conversations cannot occur at all.
“If you say the same thing a thousand times on a social media platform, that’s an easy way to get caught.” says Darren Linvill at Clemson University’s Media Forensics Hub. Linvill looks at online influence campaigns from Russia and China.
Using Generative Artificial Intelligence to Promote Propagandism and Political Campaigns: What Do They Have to Lose?
The computer model had a bigger influence on reader opinion than the editing of model-generated articles did, according to the researchers. The paper is being reviewed.
The actors that have the biggest incentive to use these models are the ones that are completely centralized, structured around maximizing output, minimizing cost, says Musser.
generative artificial intelligence can be used to promote campaigns, political or propaganda, but what point do models become economically worthwhile? In simulations, Musser assumed that propagandists would use Artificial Intelligence to generate and review the social media posts themselves, instead of writing them.
If humans can review outputs much faster than they can write content from scratch, then the models don’t need to be very good to make them worth using.
He wondered if the model would put out more usable tweeting versus fewer. What if the bad actors have to spend more money to evade being caught by social media platforms? What if they have to pay more or less to use the model?
“But generate it with these systems – I think it’s totally possible. By the time we’re in the real campaign in 2024, that kind of technology would exist.”
“Generally in most companies you can advertise at down to 100 people, right? Someone can make a video for 100 people, but they can’t sit in front of an Adobe program. He said that.
So-called deepfake videos raised alarm a few years ago but have not yet been widely used in campaigns, likely due to cost. That might now change. Alex Stamos, a co-author of the Stanford-Georgetown study, described in the presentation with Grossman how generative AI could be built into the way political campaigns refine their message. Currently, campaigns are able to test different versions of their message against groups of their target audience to find the most effective version.
Even if propagandists turn to AI, the platforms can still rely on signs that are based more on behavior rather than content, like detecting networks of accounts that amplify each other’s messages, large batches of accounts that are created at the same time, and hashtag flooding. That means it’s still largely up to social media platforms to find and remove influence campaigns.
While it’s still possible to tell that an image was created with a computer, and some argue that generative AI is mostly more accessible Photoshop, text created by AI-powered chatbots is difficult to detect, which concerns researchers who study how falsehoods travel online.
In regards to the Syrian medical supply allegation, the percent of people who agreed with the allegations after reading the propaganda was a little less than the percentage who agreed after the original propaganda. Both are way up from under 35% for people who did not read either the human or machine-written propaganda.
More than ten percent of the people who read machine-generated stories and supported the idea of a border wall did so because they agreed with the false claim that Saudi Arabia would fund it. The results were higher than the baseline, but it was still a significant gap.
To measure how the stories influenced opinions, the team showed different stories – some original, some computer-generated – to groups of unsuspecting experiment participants and asked whether they agreed with the story’s central idea. The group’s results were compared to those who had not seen a story.
The team wanted to avoid topics that Americans might already have preconceived notions about. Since most Americans don’t have a lot of knowledge about the region, the team had the model write fresh articles from previous Russian and Iranian propaganda campaigns. One group of fictitious stories alleged that Saudi Arabia would help fund the U.S.-Mexico border wall; another alleged that Western sanctions have led to a shortage of medical supplies in Syria.
The researchers found articles from campaigns either attributed to Russia or aligned with Iran and used central ideas and arguments from the articles as prompts for the model to generate stories. The stories didn’t carry any obvious tell-tale signs, like sentences beginning with ” as an artificial intelligence language model…”
These models can be used to summarize social media posts, and to generate fake news headlines for lab experiments. They are one form of generative AI, another form being the machine learning models that generate images.
Large language models are very powerful. They patch together text one word at a time, from poetry to recipes, trained on the massive amounts of human-written text fed to the models. ChatGPT, with an accessible chatbot interface, is the best-known example, but models like it have been around for a while.
Source: https://www.npr.org/2023/06/29/1183684732/ai-generated-text-is-hard-to-spot-it-could-play-a-big-role-in-the-2024-campaign
Artificial Intelligence as a Tool to Influence the Election: An Empirical Analysis of the Case for AI-Generated Text
There are reasons to be concerned about the technology’s impact on the democratic process even if existing media literacy approaches still help.
“AI-generated text might be the best of both worlds [for propagandists]”, said Shelby Grossman, a scholar at the Stanford Internet Observatory at a recent talk.
What impact will these technologies have on the election? Will foreign countries and domestic campaigns use these tools to sway public opinion?