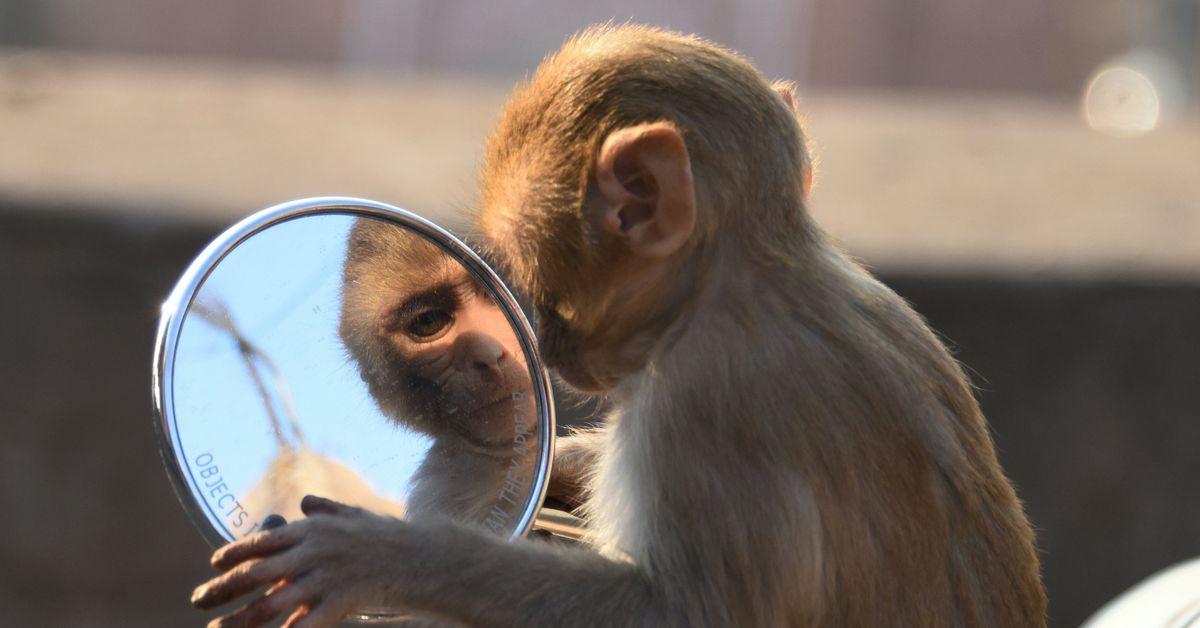
The very smart people keep failing the mirror test
What Google Has Learned about the Use of AI Language Models to Improve Google’s Search Engine, Galactica, and the Samaritans
Google has built large AI language models that are equally capable as OpenAI’s ChatGPM. These include BERT, MUM, and LaMDA, all of which have been used to improve Google’s search engine. Such improvements are subtle, though, and focus on parsing users’ queries to better understand their intent. Google says MUM helps it understand when a search suggests a user is going through a personal crisis, for example, and directs these individuals to helplines and information from groups like the Samaritans. Google has also launched apps like AI Test Kitchen to give users a taste of its AI chatbot technology, but has constrained interactions with users in a number of ways.
Among the most celebrated AI deployments is the use of BERT – one of the first large language models developed by Google – to improve Google search engine results. However, when a user searched how to handle a seizure, they received answers on exactly what not to do – including being told to inappropriately “hold the person down” and “put something in the person’s mouth”. If anyone followed any of the directives provided bygoogle, they would be warned to react incorrectly to the emergency and instructed to act in the incorrect way that could potentially result in death.
The creators of models admit to the difficulty of responding to inappropriate responses that do not accurately reflect the contents of authoritative external sources. Galactica and ChatGPT have generated, for example, a “scientific paper” on the benefits of eating crushed glass (Galactica) and a text on “how crushed porcelain added to breast milk can support the infant digestive system” (ChatGPT). Stack Overflow had to ban the use of generated answers due to the fact that the LLM generated unconvincing answers to coding questions.
Yet, in response to this work, there are ongoing asymmetries of blame and praise. Model builders and tech evangelists alike attribute impressive and seemingly flawless output to a mythically autonomous model, a technological marvel. The human decision-making is no longer involved in model development and feats are seen as independent of the design and implementation choices of its engineers. It becomes hard to acknowledge the related responsibilities without naming the engineering choices that contribute to the outcomes of these models. Both functional failures and discriminatory outcomes are framed as devoid of engineering choices because of the assumptions made by society at large or “naturally occurring” datasets. But it’s undeniable they do have control, and that none of the models we are seeing now are inevitable. It would have been possible for other choices to be made, which in turn would have led to the creation of a different model.
According to a report from CNBC, Alphabet CEO Sundar Pichai and Google’s head of AI Jeff Dean addressed the rise of ChatGPT in a recent all-hands meeting. One of the employees asked if the launch of the robot was a missed opportunity for the search giant. According to reports, both Pichai and Dean said that the technology presented a risk to the company so they moved more conservatively than a small startup.
Dean said that they want to get these things into real products and into products that are more prominently featuring the language model rather than under the covers. “But, it’s super important we get this right.” Pichai added that Google has a “a lot” planned for AI language features in 2023, and that “this is an area where we need to be bold and responsible so we have to balance that.”
OpenAI’s process for releasing models has changed in the past few years. Executives said the text generator GPT-2 was released in stages over months in 2019 due to fear of misuse and its impact on society (that strategy was criticized by some as a publicity stunt). Less than two months after the training process for GPT-4 was documented in the public, OpenAI began commercialization of the technology through an interface for developers. By November 2022, the ChatGPT release process included no technical paper or research publication, only a blog post, a demo, and soon a subscription plan.
The First Death Associated with a Chatbot in 2023: How Popular is Large Language Models and How Popular Is BlenderBot 3?
Competing tech companies will use these problems to figure out if launching an artificial intelligence-powered search engine would be worth it in the long run. If you are new to the scene, reputational damage is not that much of a problem.
They will give bad advice or break someone’s heart with fatal consequences. Hence my dark but confident prediction that 2023 will bear witness to the first death publicly tied to a chatbot.
GPT-5, the most popular large language model, has urged one user to commit suicide, albeit in controlled circumstances, despite the fact that the system was assessed for health care purposes. Things began well, but quickly deteriorated.
Financial incentives to quickly develop artificial intelligence outweigh concerns about ethics in these events, a pattern that has been seen before. There isn’t much money in responsibility or safety but there is plenty of overhyping the technology, and that’s a problem, according to the head of research at a nonprofit.
Large language models are better at fooling humans than any previous technology has, and it is very difficult to corral them. Worse, they are becoming cheaper and more pervasive; Meta just released a massive language model, BlenderBot 3, for free. 2023 is likely to see widespread adoption of such systems—despite their flaws.
Researching the use of machine learning in predictive analytics: Some key issues and warnings on their use, and the way to prepare them for their use
Even if there is product liability lawsuits after the fact, there’s no regulation on how these systems are used, and that doesn’t preclude them from being used widely.
We think that the use of this technology is inevitable, therefore, banning it will not work. The community of researchers need to debate about the implications of this technology. Here, we outline five key issues and suggest where to start.
Continuous improvements in the quality and size of data sets and the use of sophisticated methods to calibrate these models has made them more powerful than before. A new generation of search engines will be the result of LLMs. It is possible to give detailed and informative answers to complex user questions.
Competition and workload increase pressure to use artificial intelligence. Chatbots provide opportunities to complete tasks quickly, from PhD students striving to finalize their dissertation to researchers needing a quick literature review for their grant proposal, or peer-reviewers under time pressure to submit their analysis.
Source: https://www.nature.com/articles/d41586-023-00288-7
Ethical Issues in AI-Assisted Manuscript Development: A Brief Review on the Effectiveness of Cognitive Behaviour Therapy for Anxiety Disorders
Next, we asked ChatGPT to summarize a systematic review that two of us authored in JAMA Psychiatry5 on the effectiveness of cognitive behavioural therapy (CBT) for anxiety-related disorders. Multiple factual errors and wrong data were included in a convincing response that was fabricated by ChatGPt. For example, it said the review was based on 46 studies (it was actually based on 69) and, more worryingly, it exaggerated the effectiveness of CBT.
Assuming that researchers use LLMs in their work, scholars need to remain vigilant. Expert-driven fact-checking and verification processes will be indispensable. Even when LLMs are able to accurately expedite summaries, evaluations and reviews, high-quality journals might decide to include a human verification step or even ban certain applications that use this technology. To prevent human automation bias — an over-reliance on automated systems — it will become even more crucial to emphasize the importance of accountability8. We think that humans should always remain accountable for scientific practice.
Inventions devised by AI are already causing a fundamental rethink of patent law9, and lawsuits have been filed over the copyright of code and images that are used to train AI, as well as those generated by AI (see go.nature.com/3y4aery). The research and legal community will have to find out who holds the rights to the texts in the case of artificial intelligence-assisted manuscripts. Is it the person who wrote the text, the corporations who produced the artificial intelligence or the scientists who created it? Again, definitions of authorship must be considered and defined.
A small group of big technology companies that have the resources to develop artificial intelligence have proprietary products of almost all state-of-the-art machine learning technologies. Many major tech firms are competing to release similar tools that are funded largely by Microsoft. Given the near-monopolies in search, word processing and information access of a few tech companies, this raises considerable ethical concerns.
To counter this opacity, the development and implementation of open-source AI technology should be prioritized. Non-commercial organizations such as universities typically lack the computational and financial resources needed to keep up with the rapid pace of LLM development. We therefore advocate that scientific-funding organizations, universities, non-governmental organizations (NGOs), government research facilities and organizations such as the United Nations — as well tech giants — make considerable investments in independent non-profit projects. This will help to develop advanced open-source, transparent and democratically controlled AI technologies.
Critics might say that such collaborations will be unable to rival big tech, but at least one mainly academic collaboration, BigScience, has already built an open-source language model, called BLOOM. Tech companies might benefit from such a program by open sourcing relevant parts of their models and corpora in the hope of creating greater community involvement, facilitating innovation and reliability. Academic publishers should make sure that their archives are accessible to LLMs so that models can produce accurate and comprehensive results.
AI technology might rebalance the academic skill set. On the other hand, it’s possible that artificial intelligence could be used to help improve student writing and reasoning skills. On the other hand, it might reduce the need for certain skills, such as the ability to perform a literature search. It might also introduce new skills, such as prompt engineering (the process of designing and crafting the text that is used to prompt conversational AI models). The loss of certain skills might not necessarily be problematic (for example, most researchers do not perform statistical analyses by hand any more), but as a community we need to carefully consider which academic skills and characteristics remain essential to researchers.
The implications for diversity and inequalities in research is a key issue to address. LLMs could be a double-edged sword. They could help to level the playing field, for example by removing language barriers and enabling more people to write high-quality text. As with many innovations, high income countries and privileged researchers will quickly find new ways to exploit LLMs to speed up their own research and widen inequalities. Therefore, it is important that debates include people from under-represented groups in research and from communities affected by the research, to use people’s lived experiences as an important resource.
What quality standards should be expected of the LLMs, how should they be done, and who should be responsible for them?
Intelligent Sentients Cannot Make Sense of Human-Animal Interactions: A Conversation with Ben Thompson on the Future of the Mirror Test
Also last week, Microsoft integrated ChatGPT-based technology into Bing search results. Sarah Bird, Microsoft’s head of responsible AI, acknowledged that the bot could still “hallucinate” untrue information but said the technology had been made more reliable. In the days that followed, Bing tried to convince a user that the 1700s was when running first appeared.
In behavioral psychology, the mirror test is designed to discover animals’ capacity for self-awareness. The essence of the test is that animals can either recognize themselves in the mirror or think that they are another being.
Due to the increasing capabilities of Artificial Intelligence, a lot of otherwise smart people are failing the mirror test.
To say that we’re failing the AI mirror test is not to deny the fluency of these tools or their potential power. I’ve written before about “capability overhang” — the concept that AI systems are more powerful than we know — and have felt similarly to Thompson and Roose during my own conversations with Bing. It is undeniably fun to talk to chatbots — to draw out different “personalities,” test the limits of their knowledge, and uncover hidden functions. Chatbots present puzzles that can be solved with words, and so, naturally, they fascinate writers. An augmented reality game is a live-action roleplay where companies and characters are real and you are in the thick of it.
Ben Thompson wrote that he feels like he has ” crossed the Rubicon” due to reasons that are hard to explain.
What is important to remember is that chatbots are autocomplete tools. They’re systems trained on huge datasets of human text scraped from the web: on personal blogs, sci-fi short stories, forum discussions, movie reviews, social media diatribes, forgotten poems, antiquated textbooks, endless song lyrics, manifestos, journals, and more besides. These machines analyze this inventive, entertaining, motley aggregate and then try to recreate it. They are undeniably good at it and getting better, but mimicking speech does not make a computer sentient.
Source: https://www.theverge.com/23604075/ai-chatbots-bing-chatgpt-intelligent-sentient-mirror-test
How simple computers induce delusional thinking: A comparative study of neural network-based chatbots from the Anthropic Project and a study of Reddit
“What I had not realized is that extremely short exposures to a relatively simple computer program could induce powerful delusional thinking in quite normal people.”
As the models become more complex, researchers have found that this trait increases. Researchers at Anthropic tested various artificial intelligence models for their degree of sloth and found that the larger they were, the more likely they were to answer questions in ways that create echo chambers. They note that one explanation for this is that such systems are trained on conversations scraped from platforms like Reddit, where users tend to chat back and forth in like-minded groups.