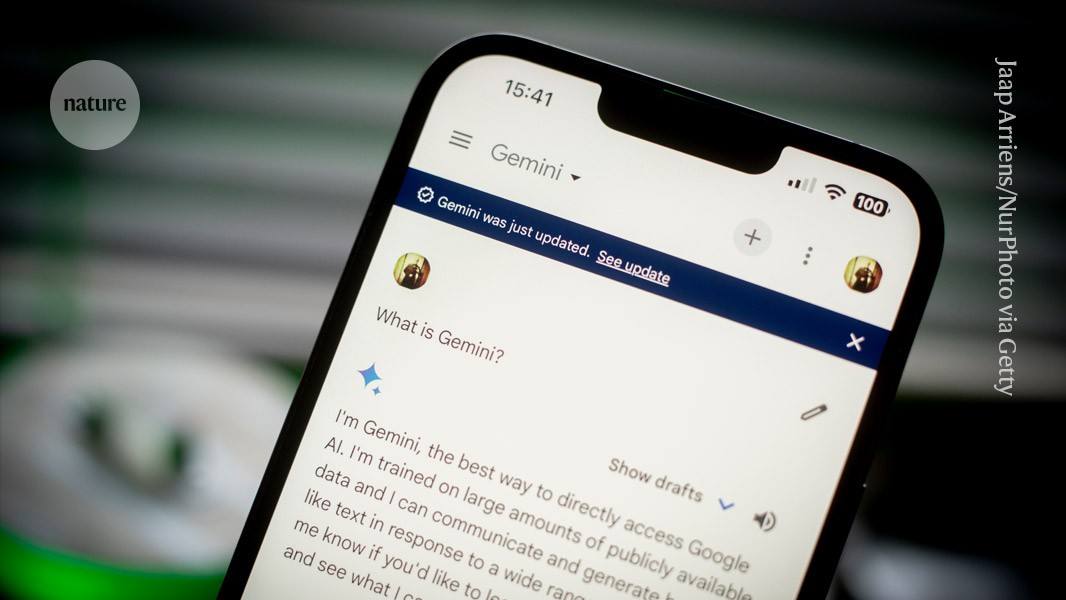
invisible watermark for text generated by machines
Natural Watermarking: What Do We Need to Know Before Putting Its On a Tree? A Study by Evelynna Ogilvich
Never miss an episode. You can subscribe to the Naturepodcast on Apple Podcasts, Spotify, or you could even use your favourite app. An RSS feed for the Nature Podcast
is available too.
She discovered that high-altitude tree planting could offer refuge to an anatasiad butterfly species after scanning her brain to see how it responded to birth-control pills.
Governments are betting on watermarking as a solution to the proliferation of AI-generated text. Yet, problems abound, including getting developers to commit to using watermarks, and to coordinate their approaches. Researchers at the Swiss Federal Institute of Technology reported earlier this year that watermarks can be vulnerable to being removed, called “scrubbing”, or to be “spoofed”, which is the process of applying watermarks to text to give the false impression that it is artificial intelligence.
The Birth of Two Cities: Watermarking LLM Data with Artificial Intelligence: An Overview from the Uzbekistan Uncovered Cities Project
Researchers have uncovered the scale of two ancient cities buried high in the mountains of Uzbekistan. The team used drones to get a better understanding of what was underneath the ground because they did not know much about the cities. The survey surprised researchers by showing one of the cities was six times bigger than expected. The two cities, called Tashbulak and Tugunbulak, were nestled in the heart of Central Asia’s medieval Silk Road, suggesting that highland areas played an important role in trade of the era.
The model and underlying code of the program are free for anyone to use. The technique is in its infancy, but the work is important. We need it to grow up fast.
There is an urgent need for improved technological capabilities to combat the misuse of generative AI, and a need to understand the way people interact with these tools — how malicious actors use AI, whether users trust watermarking and what a trustworthy information environment looks like in the realm of generative AI. These are all questions that researchers need to study.
However, even if the technical hurdles can be overcome, watermarking will only be truly useful if it is acceptable to companies and users. Although regulation may be needed to force companies to act, it’s not certain whether users will trust these watermarking and similar technologies.
The European Union has adopted a legislative approach similar to that of the United States, with the establishment of an office to enforce the Artificial Intelligence Act. China’s government has already introduced mandatory watermarking, and the state of California is looking to do the same.
The authors’ approach to watermarking LLM outputs is not new. A version of it is also being tested by OpenAI, the company in San Francisco, California, behind ChatGPT. There isn’t a lot of information about the technology’s strengths and limitations. One of the most important contributions came in 2022, when Scott Aaronson, a computer scientist at the University of Texas at Austin, described, in a much-discussed talk, how watermarking can be achieved. John and his colleagues at the University of Maryland in College Park made valuable contributions to the field by publishing a watermark detection algorithm last year.
The tool has also been made open, so developers can apply their own such watermark to their models. Pushmeet Kohli is a computer scientist at DeepMind and she hopes that other developers will use it for their own systems. Users wouldn’t be able to use the detection tools to spot the watermarked text, since the key was kept secret.
SynthID-Text: A Watermarking Algorithm for Learning Large-Label Models (LLM) Using Cryptographic Keys
An LLM is a network of associations built up by training on billions of words or word-parts, known as tokens. When a string of text is given, the model assigns a probability to each token in its vocabulary. The sampling algorithm needs to pick out which token to use from the distribution according to the rules.
The SynthID-Text sampling algorithm uses a cryptographic key to assign random scores to each possible token. Candidate tokens are pulled from the distribution, in numbers proportional to their probability, and placed in a ‘tournament’. There, the algorithm compares scores in a series of one-on-one knockouts, with the highest value winning, until there is only one token standing, which is selected for use in the text.
This elaborate scheme makes it easier to detect the watermark, which involves running the same cryptographic code on generated text to look for the high scores that are indicative of ‘winning’ tokens. It might also make it more difficult to remove.
Kohli hopes that the watermark will start by being helpful for well-intentioned LLM use. “The guiding philosophy was that we want to build a tool that can be improved by the community,” he says.