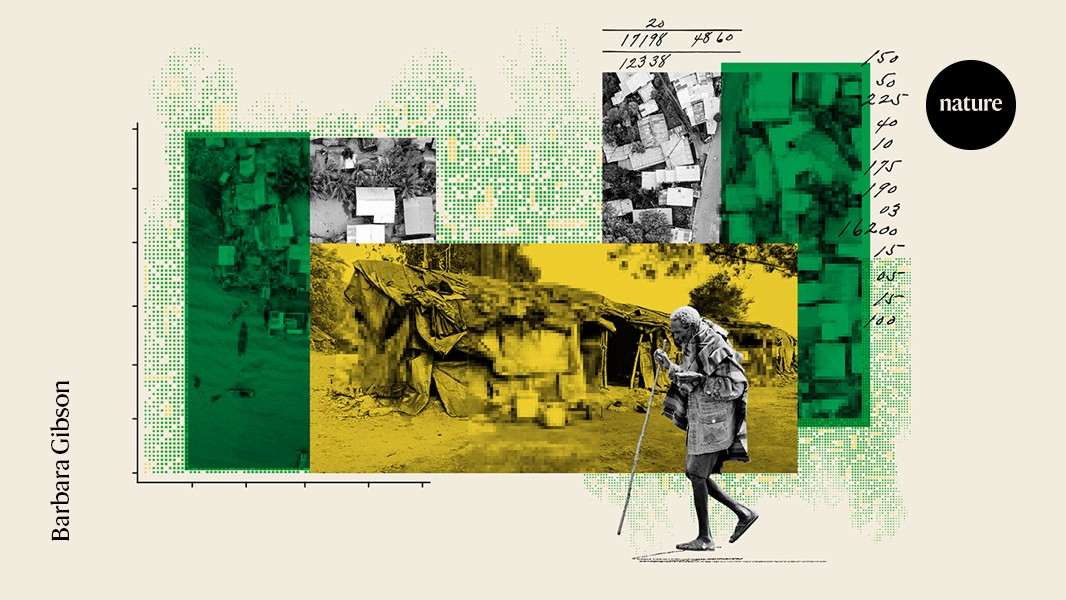
Researchers are trying to see whether Artificial Intelligence can be used to help poor people
The problem of time and space: a human geography student’s look at AI-driven data collection, which could help public research in areas of poverty
Artificial-intelligence tools and community science can help in places where data are scarce, so long as funding for data collection does not falter in the future.
There are some reasons to be cautious, says human geography student at Lund University, who is researching the intersection of poverty and artificial intelligence. People have criticized the models for being biased. Just as household surveys often miss the poorest families because they do not have permanent housing, AI-driven programmes might not help individuals who do not have digital data trails, Hall says. He says that they are not Accurate enough to determine who qualifies for aid or cash subsidies.
Along with the lack of agreement on definitions, there’s the problem of time. Jolliffe says a field team needs several hours to survey a single family. Poverty researchers are refining their metrics and using new methods for analyzing data but they still use on-the-ground surveys to collect the data. Jolliffe says people are surprised that we do household surveys. The idea that we have data on every single person is a rich-world perspective.
Could artificial intelligence be able to offer a solution? The short answer is, yes, although with caveats. The Togo example shows how AI-informed approaches helped communities by combining knowledge of geographical areas of need with more-individual data from mobile phones. It’s a good example of how AI tools work well with granular, household-level data. Citizen scientists, also known as community scientists, are a relatively undiscovered source of such information. This idea deserves more attention and more funding.
Thanks to technologies such as smartphones, Wi-Fi and 4G, there has been an explosion of people in cities, towns and villages collecting, storing and analysing their own social and environmental data. In Ghana, for example, volunteer researchers are collecting data on marine litter along the coastline and contributing this knowledge to their country’s official statistics.
Last December a group of data scientists argued in an article that data could be utilized by policymakers as well as with the use of artificial intelligence. Nature supports itself. 8, 125–132, 25 years later. In the piece, Dilek Fraisl and colleagues are calling for a partnership between citizen scientists and artificial intelligence researchers.
The authors could be pushing at an open door. International organizations such as the United Nations Statistical Commission, which sets the standards for measuring official statistics, want more citizen scientists to contribute data, such as for the UN Sustainable Development Goals (SDGs), the world’s plan to end poverty and achieve environmental sustainability. The UN sees citizen science and citizen data as a potential solution for having poor representation of hard-to-reach populations in progress reports.
Evaluating Poverty: How Charles Booth and his colleagues built a Unified Indice to Address Vulnerabilities in the Age of AI
AI has to be deployed in a way that maximizes benefits and mitigates or reduces risks. This is especially important when it comes to using AI that involves people who are vulnerable or living in poverty. Artificial intelligence has to make their lives better and not expose them to harms.
AI allowed Lawson to leapfrog the conventional hurdles of using old and incomplete data to quickly make the most of her limited budget. It’s an approach that is garnering both interest and controversy, says Joshua Blumenstock, a computer scientist at University of California, Berkeley, who collaborated on Novissi.
However flawed AI might be, though, current systems of evaluating poverty are just as abysmal, says BenYishay. “The baseline isn’t perfect data. It’s actually very crappy data,” he says.
British social reformer Charles Booth undertook an early effort to quantify poverty from 1886 to 1903 when he criss-crossed London’s cobblestones collecting data on people’s incomes and social class. He created a map of the city with a colour code, and wrote about it in a book. Rowntree and his team wrote a 1901 book about poverty, called Poverty: A Study of Town Life. The team calculated poverty on the basis of the ability to meet a person’s “physical efficiency”, or their minimal nutritional requirements. A sample minimal diet might include bread, porridge, boiled bacon, potatoes, skimmed milk and little else.
The Office of Economic Opportunity adopted a poverty threshold after Lyndon Johnson declared a War on Poverty in 1964. It defined poverty as the bare minimum income required to cover food, shelter and other basic costs. Around the same time, India performed similar calculations for its populace. Poverty was determined by how much money a person has per day, rather than by rent or food costs, which were all accounted for in each expert’s formulae.
Alkire wanted a way to study the effects of poverty on people. Just because someone has enough money to buy food doesn’t mean they have enough for medical care or school fees, says Alkire. In 2008, Alkire and James Foster worked together to create the Multidimensional Poverty Index. The approach estimates a unified measure of poverty by tallying up the deprivations and their intensity, with a total of ten indicators, including nutrition, school attendance, access to drinking water and what a household uses for cooking fuel.
“This was a pretty seminal improvement concept for the development community,” says BenYishay. Other teams are joining the experiment, throwing out a lot of different ideas, he says. There are methods that are being used to look for patterns in satellite images, as well as to look at impacts on agriculture, infrastructure investments and more.