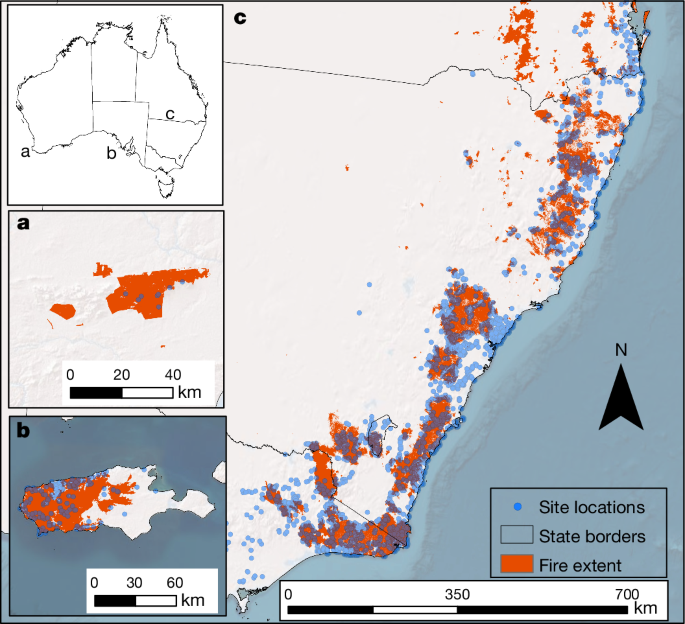
The impacts of megafires in Australia
Modelling the consequences of the Australian megafires for biodiversity using a t-test with denominator degrees of freedom adjusted for random effects
It seemed like everything was burning down for a while. In California, Oregon and Washington, fire records were destroyed in 2020 and 2021, with a devastating effect on wildlife habitat. In 2020, 4 million hectares of drought-stricken wetland and savannah burnt in the Brazilian Pantanal region, killing 17 million animals3. In late 2019 and early 2020, bushfires raged during the height of summer in Australia, burning an astonishing 10.3 million hectares of shrubland and forest, corresponding to just under half the area of the United Kingdom. Writing in Nature, Driscoll et al.4 present a detailed look at the consequences of the Australian megafires for biodiversity.
The conclusions and findings in the essay are of the author and should not be used to represent official USDA or US Government policy.
Where there was no evidence for an interaction between a covariate and fire severity (P > 0.1) we applied a univariate model with the covariate as the only moderator. In these cases, we used the effects calculated using unburnt versus burnt sites rather than unburnt versus sites burnt at either high or low severity. The analysis was the same, except without the need for a variance–covariance matrix, because unburnt sites were only used once.
We put the models into the rma.mv function of the metafor. The package is called R72. The Nelder–Mean method of model maximization gave us restricted maximum likelihood 73. We used a t-test with denominator degrees of freedom adjusted for random effects using the ‘contain’ option63,73.
To evaluate the effects between years, the dataset was divided into two periods: Year 1 and Year 2. We were able to define surveys that were taken up to 1.5 years after fire and surveys that were taken up to 2 years after fire. We used the time gaps in survey dates to maximize the data that could be included for each project, with the compromise that this led to minor temporal overlap of our year 1 and year 2 effects (one Year 2 project started in February 2021; two Year 1 projects ended in June 2022). Ten datasets had 1–5 months of data excluded (Extended Data Table 3).
Before using the MC and MD functions, data for species within projects were standardized by dividing all values by the maximum value for that species within that project, so that the data for calculating each effect size ranged from zero to one. While this makes data with different ranges of values comparable, it also risks biasing down effect sizes when there are large outliers. We therefore also calculate MC and MD after removing outliers. We used an approximation that y is usually distributed with a s.D. of and defined outliers as those greater than the median. This approach is conservative, such that if the Poisson lambda value is less than three, it will identify fewer outliers. It doesn’t identify outliers when applied to binomial data. The results were similar to when outliers were included, but the effect sizes were slightly larger.
The Third Design of the After-Control-Impact Control for Long-Range Heavy Ion Collisions at BNL RHIC
The third design was included in the after-control-impact projects. The design was converted to control by subtracting the after-fire response for each site. Subsequently, the SMD was calculated using the same method as the second design.