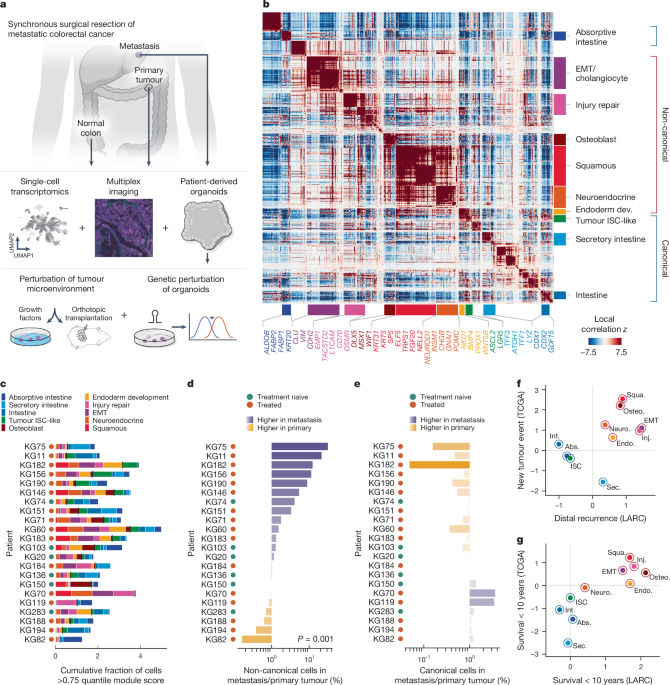
The colorectal cancer metastases have progressive plasticity
GSEA in normal and tumour epithelial cells: analysis of the first lognormalized expression matrix using the Xenium Panel Designer
We took a subset of the single-cell dataset containing all untreated normal and tumour epithelial cells (13,935 cells) and performed PCA of the log-normalized expression matrix. We focused on the first PC (PC1), which we note was responsible for 13.5% of the variance in the dataset (compared to 8.96% of variance described by PC2). To order all genes according to their features on PC1, we only ordered genes with zero-valued loadings. Using this ordering, we performed GSEA using the prerank function of the Python package gseapy (v.0.14.0) with relevant cell type gene sets from the literature13,21,61,64,65 (Supplementary Table 3) and the default parameters as inputs (Fig. 3d).
You can use the Xenium Panel Designer to create your own custom genes or probes, following the instructions in the’Getting Started with Xenium Panel Design’ instructions. In brief, 21-bp sequences flanking the targeted transcribed variant site were curated from the Ensembl canonical transcript (Ensembl v.100). All four possible ligation junctions (two for the WT allele and two for the variant allele, three in the case of deletions—two for the WT allele and one for the variant allele) were then evaluated. Variant sites for which only non-preferred junctions (CG, GT, GG and GC) were available were excluded. The two bases of the ligation junction sequence were the last base of the RBD5 (RNA binding domain) and the first base of the RBD3 probe. Preferred junctions were always prioritized over neutral junctions unless a neutral junction was necessary to avoid hairpins, homopolymer regions, dimers or an unfavourable annealing temperature. Probe lengths for RBD5 and RBD3 were then adjusted from the 21-bp starting length to target a temperature between 50 °C and 70 °C per probe (overall target 68 °C and 82 °C). The sites that were predicted to form hairpins or dimers by the analyzer were not included. The sites with more than one consecutive base in both the RBD5 andRBD3 probes were not included.
Determination of 3D Tumour Microregions Using a 3D Margins Algorithm with a Multistep Process Using H&E and Visium ST
We then use a gating procedure to identify cell types. To combat the differences of intensity distributions between tissue types and runs, thresholds were set manually for every channel in the cell. Above this intensity threshold, a pixel was considered positive for a given marker, and below it, a pixel was considered negative. From the boundaries of the cells, we used to calculate the cell typing markers in each cell. The result of this process is a feature matrix (num cells × num proteins) representing positive marker fractions for each cell typing protein in every cell. A cell was considered positive for a marker if >5% of its pixels were positive for that marker. Cells were then labelled with a gating strategy specific to each sample. Each cell was subjected to an AND gate, where if they passed all criteria, it was annotated as the cell type specified for the step, whereas if they failed, it was passed on to the next step. The gating strategies used for the samples are in Supplementary Table 4.
A new volume was built where neighbourhoods which used the above criteria were considered to be tumour neighbourhoods, and all other voxels were not. This 3D tumour mask was then smoothed with a Gaussian kernel (sigma = 1.0). The values were used as input to create a surface mesh for the tumours. The implementation of the marching cubes algorithm was used with default parameters.
Using Visium ST, tumour microregions were determined through a multistep process using H&E. Each ST spot was assigned as either stroma or tumour by manually reviewing the morphology on H&E stained sections. If at least 50% of the pixels within a spot covered malignant cell morphology, the spot was labelled as tumour. Otherwise it was called stroma. Next, we defined distinct tumour microregions using a set of three rules. The first rule says that tumour spots immediately adjacent to one another should be marked as a single tumours microregion. The second rule states that if two distinct tumour regions together occupied at least 50% of one single spot, the spot is assigned to the distinct tumour region with the higher percentage occupied. Finally, the third rule specified that if there was a clear morphological difference of the tumour spots within one tumour microregion, the microregion must be separated into distinct microregions, one per clear morphology.
Our workflow for cell annotation consisted of four main steps: (1) image format conversion, (2) cell segmentation, (3) spatial feature generation and (4) cell-type classification. We made the CODEX platform’s image output compatible with the open-sourced OME-TIFF format. During this process, we also produced a separate image for each sample, as multiple sections of tissue are sometimes included on the same imaging run. The Mesmer model is used to segment cells in the Deep cell framework75. DAPI was used as the nuclei intensity image, and the channels pan-cytokeratin, HLA-DR, SMA, CD4, CD45, Hep-Par-1, CD31, E-cadherin, CD68 and CD3e were, for those present in a given image, mean-averaged to a single channel and used as the membrane intensity image.
The subclone boundary region for tumour clones was defined as the union of the outermost layer of subclone annotated spots and the spots one layer expanded out from them, representing an area roughly 100–150 µm at the tumour–TME interface. Subclone-specific fractions were calculated as the neighbourhood overlaps with the outermost layer of each subclone.
We sorted out neighbourhoods with more than 50% overlap with copy number annotated subclones to focus on neighbourhoods most related to the TME biology. We did not include neighbourhoods that mapped to fewer than ten total spots across all sections for a sample.
Neighbourhoods were then assigned to Visium ST spots in the following manner. Each spot had a label assigned to it by the neighbourhood.
A graph-based clustering approach has been used to generate multiple data-type specific volumes for the neighbourhood volumes. In brief, all overlapping neighbourhood voxel annotations were identified. A graph was then constructed, whereby nodes represented each neighbourhood partition combination, and edges are the distance (in the expression profile) between these partition combinations. This graph was then clustered with the Leiden graph clustering algorithm to identify integrated neighbourhoods. The above clustering process hasparameters in Supplementary Table 4. 3D neighbourhoods were displayed using an open-sourced visualization tool.
After the assignment of neighbourhoods for each section, slides were interpolated to generate a 3D neighbourhood volume. The torchio library74 gave us linear interpolations of neighbourhood assignment probabilities.
Following training, the model inference was performed on overlapping image tiles for each slide using a sliding window of size 8 and a stride of 2 (that is, 2 overlapping patches between image tiles). Retiled patches were taken from the centre of each tile to match the original slide orientation. Each patch was at a resolution of 50 m–1 with an exception of Visium ST, which had a patch resolution of 100 m–1.
Two separate runs of the model were trained to be used for the other two slides. The table contains training hyperparameters such as the amount of training steps and the amount of batches. Only one instance was trained because the data type wasn’t present. Three model instances were trained and merged at the end of the process described in the section 3D neighbourhood construction and integration.
Where λNBHD (maximum of 0.01) and λMSE (set to 1.0) are scalers for the neighbourhood loss (LNBHD) and reconstruction loss (LMSE), respectively. During training, λNBHD for was linearly increased from 0 to its maximum value.
Source: Tumour evolution and microenvironment interactions in 2D and 3D space
Neighbour assignment in a codebook-based autoencoder for multi-level deep inelastic image reconstruction by the ViT
$${{\bf{L}}}{{\rm{o}}{\rm{v}}{\rm{e}}{\rm{r}}{\rm{a}}{\rm{l}}{\rm{l}}}={\lambda }{{\rm{N}}{\rm{B}}{\rm{H}}{\rm{D}}}{{\bf{L}}}{{\rm{N}}{\rm{B}}{\rm{H}}{\rm{D}}}+{\lambda }{{\rm{M}}{\rm{S}}{\rm{E}}}{{\bf{L}}}_{{\rm{M}}{\rm{S}}{\rm{E}}}$$
During the course of training, the autoencoder tried to align the neighbourhood labels between the adjacent sections while reconstructing the expression profile of each image patch. Both objectives forced the model to learn representative expression patterns, as well as keeping neighbourhoods aligned between input section, which helped to combat neighbourhood differences. There were differences in patch expression quantified by theMSE, whereas neighbourhood adjacency was enforced by minimizing the cross-entropy of patches nearby each other.
The overall loss function has two main contributions: mean squared error (MSE) on the reconstruction of the input patches, and cross-entropy loss on the encoded distribution and the normal distribution with 0 mean and 1.0 variance.
The patches were represented as an embedded of size n d. The next step in the architecture was neighbourhood assignment. The neighbourhoods were assigned to patches based on their levels of specificity, with several neighbourhoods being classified into different neighbourhoods. For each level of the neighbourhood hierarchy, the subsequent levels comprised partitions of the previous levels’ neighbourhoods, that is, except for the first level, each neighbourhood was a subset of a neighbourhood in a previous level of the hierarchy. The model generated three levels of neighbourhoods, each with a capacity to discover up to 64 neighbourhoods. For this analysis, all neighbourhoods shown are neighbourhoods annotated at hierarchy level 3. The model contained three codebooks (one for each level) that are of size n_NBHDs × d, where n_NBHDs is the number of possible neighbourhoods that can be assigned for the given level. The patch embeddings output by the ViT encoder were projected by three independent blocks of linear layers (one for each level) that output each patch’s probability of assignment to a given neighbourhood. These probabilities were then used to retrieve neighbourhood embeddings from the codebook corresponding to the neighbourhood level. Three linear blocks (one for each level) were then used to independently reconstruct patch embeddings at each level to each patch’s original pixel values. The code used for training the model is available at GitHub (https://github.com/ding-lab/mushroom/tree/subclone_submission).
ViTs work on image tokens as input. An image token is a representation of the patches of the input image. During training, image tiles were sampled from a uniform distribution across the set of input sections (Supplementary Fig. 7a). The sampled tile was then split into patches, for which the number of patches was determined by two hyperparameters: patch height (ph) and patch width (pw). Each patch was then flattened to a 1 × (ph × pw × c) vector, where c is the number of channels in the image (in the case of spatial transcriptomics data, c is the number of genes). The unrolled patches were then concatenated into a n × (ph × pw × c) matrix, where n is the number of patches in the image tile. Each row in this matrix is a token that represents a patch in the image tile. The tokens were then projected by a linear layer to shape n × d, where d is the dimension of the transformer blocks.
Expression profiles for each patch were generated differently for image-native data (CODEX and H&E) and point-based data (Visium). The average of the image channels within the patch bounds was used to calculate expressions for CodeX and H&E patches. Visium patches were calculated in largely the same manner; however, the expression profile of each spot within the patch was linearly weighted by its distance to the centre of the patch. The differential weight helped to account for the number of spots that fall within the patch boundaries.
We used BigWarp 71 in the application for registration. To register each collection of serial images, we used the first serial section as the fixed image and the second image as the moving image. The second image was used as a fixed image to transform the first image into the third image. The serial section experiment had a key point registration for all images. A total of 4–20 key points were selected per image transformation. Each image transformation was exported from Big Warp after key points were selected. This dense displacement field was then upscaled by a factor of 5 so it could be used to warp the full-resolution imaging data. The full-resolution dense displacement field was then used to register its corresponding multiplex or Visium data. The code used for registration is available at GitHub (https://github.com/ding-lab/mushroom/tree/subclone_submission).
Before registration, imaging data underwent the following transformations. Multiplex images were converted to greyscale images of DAPI intensity. The image was then downscaled before key point selection. H&E images (also downsampled by a factor of 5) were used for keypoint selection with Visium data.
We evaluated the spatial-based cell–cell interaction (CCI) in the ST sample using COMMOT69 with CellChat database and distance threshold of 1,000 µm, following the same threshold used in the original publication for Visium. The signal from the sender and receiver was compared with the signal from the non-boundary spots on a sample. Interaction pathways with signal difference great than 0.1 and FDR less than 0.05 are considered significantly boundary-enriched. Boundary DEGs were discovered using the FindMarkers function on three sets of comparisons. In the boundary test, a boundary DEG has adjusted P value 0.25 to correspond with the other two tests.
To distinguish transcripts originating from cancerous versus non-malignant stromal or immune cells, we used merged snRNA-seq data per organ (breast, kidney, liver and pancreas) for cell-type marker analysis. The results were obtained using the FindAllMarkers function in Seurat. We used filters like average log2(fold change) > 2, per cent expression in at least one cell type to ensure robust marker selection for each cell type. The list of genes is in Supplementary Table 5. This list was very useful for removing non-cancerous cell genes from analysis of cancer specific expression patterns. When using the RCTD de convolution method, we observed a mapping of all the different types of cells. This observation probably stems from the diverse BRCA subtypes present in the cohort. We combined all cells into a single category during the identification of cell-type markers and excluded them from the blacklist. For tumours originating from organs other than the four mentioned above, we aggregated all genes present in the blacklist across organs to form a comprehensive multiorgan blacklist, which aided in filtering out non-cancerous transcripts.
In the same fashion, rank statistics were calculated for each test as –log10(P value) × rho for tests with P < 0.05. The rank was 0 for tests with P 0.05 or genes not tested. We then calculated average rank statistics per case, followed by the average per cancer type. Finally, with the full list of rank statistics calculated for all genes tested, we used the function GSEA (parameters: pvalueCutoff=0.5; package: clusterProfiler v.3.18.1) to obtain the normalized enrichment score of Hallmark pathways (package: msigdbr 7.5.1) from the MSigDB64. Finally, only pathways with P < 0.1 were kept in the final results.
There are ten cases that we focused on and we had multiple spatial subclones for this analysis. We used FindMarkers from Seurat to get DEGs between each subclone and TME. The cut-off was applied for the P 0.01, average log2(fold change) > 1 and per percent expression in at least one cell type to select significant DEGs. To infer treatment response, we used the perturbation database LINCS L1000 (ref. 65), specifically the LINCS_L1000_Chem_Pert_down dataset from Enrichr66, to evaluate the gene set overlap between upregulated DEGs in spatial subclones and downregulated genes after compound treatment. The data was sorted by the name of the subclone and then the top compounds were selected. The mechanism of action and associated compound Metadata were taken from Clue.io to get the heatmap annotations.
The reverse applied to candidate stromal-specific DEGs. If a DEG did not meet both of these requirements to be tumour or stromal specific, it was designated as either tumour-enriched or stromal-enriched based on whether the expression level was higher in tumour or stromal cell types (Supplementary Fig. 8b).
Cell-type assignment was done based on the following known markers: B cell, CD79A, CD79B, CD19, MS4A1, IGHD, CD22 and CD52; cDC1, CADM1, XCR1, CLEC9A, RAB32 and C1orf54; cDC2, CD1C, FCER1A, CLEC10A and CD1E; mregDC, LAMP3, CCR7, FSCN1, CD83 and CCL22; pDC, IL3RA, BCL11A, CLEC4C and NRP1; macrophage, CX3CR1, CD80, CD86, CD163 and MSR1; mast cell, HPGD, TPSB2, HDC, SLC18A2, CPA3 and SLC8A3; endothelial, EMCN, FLT1, PECAM1, VWF, PTPRB, ACTA2 and ANGPT2; fibroblast, COL1A1, COL3A1, COL5A1, LUM and MMP2; pericyte, RGS5, PLXDC1, FN1 and MCAM; NK cell, FCGR3A, GZMA and NCAM1; plasma cell, CD38, SDC1, IGHG1, IGKC and MZB1; T cell, IL7R, CD4, CD8A, CD8B, CD3G, CD3D and CD3E; and regulatory T cell, IL2RA, CTLA4, FOXP3, TNFRSF18 and IKZF2. Normal epithelial cells in the breast were annotated with the following markers: LumSec, GABRP, ELF5, CL28, KRT15, BARX2 and HS3ST4; LumHR, ANKRD30A, ERBB4, AFF3, TTC6, ESR1, NEK10 and XBP1; and basal, SAMD5, FBXO32, TP63, RBBP8 and KLHL13. Normal epithelial cells in the liver were annotated with the following markers: hepatocyte, ALB, CYP3A7, HMGCS1, ACSS2 and AKR1C1; cholangiocyte, SOX9, CFTR and PKD2. Normal epithelial cells in the pancreas, including ductal, acinar, islet-α, islet-β and islet-γ cells, were annotated with singleR (v.1.8.1) using reference data BaronPancreasData(‘human’).
Estimation of microregion density per section in the visium gel v1 gene expression slide by spatial resolution and configuration of the capture area
We estimated microregion density per section by following the formula: density per µm2 = n microregion per section size (in spots) then divided by 8,660 µm per spot. Then density per mm2 = density per µm2 × 106 (n microregion per mm2).
To calculate the area each spot takes, we used the spot size (55 µm) and centre-to-centre distance between each spot (100 µm) provided by 10x Genomics (http://kb.10xgenomics.com/hc/en-us/articles/360035487572-What-is-the-spatial-resolution-and-configuration-of-the-capture-area-of-the-Visium-v1-Gene-Expression-Slide-). As illustrated in Supplementary Fig. 6, the Visium spots form a hexagonal lattice that covers the sample. Each spot in the lattice has a shape that is composed of eight equilateral triangles. Each triangle has a side of 50 µm (half of the spot the centre-to-centre distance). Using the area equation of equilateral triangles and multiplying it by 8, we obtained the area of each trapezoid as 8,660 µm2, which is the average area occupied by each spot. To get the microregion size, we took the spot count and divided by 106 to get a total.
Indicates the size of the genomic window i and the CNV annotations in profile A.
It’s sim’s size and times.
Source: Tumour evolution and microenvironment interactions in 2D and 3D space
Analysis, visualization, and integration of somatic CNVs with GATK: A Seurat vignette for spatial data analysis and modelling of tumours
Somatic CNVs were called using GATK (v.4.1.9.0)61. Specifically, the hg38 human reference genome (NCI GDC data portal) was binned into target intervals using the PreprocessIntervals function, with the bin length set to 1,000 bp and using the interval-merging-rule of OVERLAPPING_ONLY. Each normal sample was used to input the GATK functions CollectReadCounts, and the minimum-Interval-median argument was used to create a panel of normals. For tumour samples, reads that overlapped the target interval were counted using the GATK function CollectReadCounts. Tumour read counts were then standardized and denoised using the GATK function DenoiseReadCounts, with the panel of normals specified by –count-panel-of-normals. According to GATK best practices, allelic counts for tumours were created using the GATK function collect alleliccount. The model segments were made using the GATK function ModelSegments and denoised copy ratio as inputs. GATK uses a function to call copy ratios on segments.
For each sample, we obtained the unfiltered feature–barcode matrix per sample by passing the demultiplexed FASTQ files and associated H&E image to Space Ranger (v.1.3.0, v.2.0.0 and v2.1.0 ‘count’ command using default parameters with reorient-images enabled) and the prebuilt GRCh38 genome reference 2020-A (GRCh38 and Ensembl 98). Seurat was used in all the analyses. We constructed a Seurat object using the Load10X_Spatial function for every slide. The slide was scaled and normalized with the functions of the SCTransform function. Any merged analysis or subsequent subsetting of cells and samples for a sample with several slides underwent the same scaling and normalization method. Spots were clustered using the original Louvain algorithm, and the top 30 principal component analysis dimensions using the FindNeighbors and FindClusters functions as described in the ‘Analysis, visualization, and integration of spatial datasets with Seurat’ vignette from Seurat (https://satijalab.org/seurat/articles/spatial_vignette.html).
Bedtools62 intersection was used to map copy number ratios from segments to genes and to assign the called amplifications or deletions. For genes that overlap multiple segments, a custom script was used to amplify, neutral or deletion based on a weighted copy number ratio and the length of the overlaps. The bounds of the default Z score thresholds were used instead, if the resultingz score cut-off value was within that range.
For each ST section, we applied two sets of statistical tests to all WES-based somatic mutations mapped to ST. First, for each mutation with greater than 30 reads of coverage on ST across all spots, the VAF was calculated for all tumour region spots and all non-tumour region spots as the number of variant reads across all spots divided by the number of total reads across all spots. The binom.test used VAF of non-tumour spots as the background. A proportion test was performed between the VAFs in different subclones. Finally, multiple testing correction was done on both sets of tests with the function p.adjust().
Tumour tissue samples were obtained from surgically resected specimens. The remaining sample was frozen in liquid nitrogen and stored at 80 C after a piece was removed for fresh preparation. Before bulk DNA extraction, samples were cryopulverized (Covaris) and aliquoted for bulk extraction. The QIAamp DNA Mini kit was used to extract the genetic material from tissue samples. A Qiagen DNA Mini kit was used to purify Genomic germline DNA from peripheral blood mononuclear cells. The DNA quantity was assessed by fluorometry using a Qubit dsDNA HS assay (Q32854) according to the manufacturer’s instructions (Thermo Fisher Scientific). There are a number of protocols at protocols.io.
Total RNA was extracted from organoids using the RNeasy Mini kit (Qiagen). The first strand of the cDNA was prepared using the Transcriptor First-Strand cDNA synthesis kit. qPCR was performed with TaqMan gene expression assay primers (Thermo Fisher Scientific; PROX1, Hs00896293_m1; GAPDH, Hs02758991_g1; ELF5, Hs01063022_m1; KRT23, Hs00210096_m1; TFF3, Hs00902278_m1; FABP1, Hs00155026_m1; TRPS1, Hs00936363_m1; GNAI1, Hs01053355_m1; FGF20, Hs00173929_m1; NELL2, Hs00196254_m1; NRXN3, Hs01028186_m1; POMC, Hs01596743_m1; KRT20, Hs00300643_m1; GPC1, Hs00892476_m1; LGR5, Hs00173664_m1; TMEM132A, Hs01096434_m1; NEUROD1, Hs01922995_s1; CHGB, Hs01084631_m1), the Relative expression was quantified using the ΔΔCt method, normalized to the expression of GAPDH on the QuantStudio 6 and 7 Pro real-time PCR system (Applied Biosystems).
Whole organoids were plated in 10%Matrigel andHISC medium on chamber slides and then put into a 37 C petri dish. The organoids were fixed in 4% paraformaldehyde in a PME solution for 10 minutes, washed twice, and then permeabilized. Organoids were incubated with 1:400 of 594 anti-rabbit antibody (Invitrogen, A11012) for 1 h at room temperature, washed three times with IF buffer and one time with cold DPBS. The wells were removed and the samples mounted using a medium containing DAPI. Imaging was performed on the Zeiss Axio Imager 2 with Apotome structured illumination microscope. PROX1 expression was quantified using the CellProfiler (v.4.2.5) software85.
Oil droplets were used to isolated chondrie and cells from barcoded beads. The range of single-nucleus suspensions was calculated using a haemocytometer. Reverse transcription was subsequently performed to incorporate cell and transcript-specific barcodes. The snRNA-sq samples were run using a Chromium Next GEM library and gel bead kit. The multiome kit used 10x Genomics and the single cell multiome was used with Chromium Next GEM. Nuclei were then subjected to downstream protocols by 10x (Next GEM Single Cell Multiome ATAC + Gene Expression: https://cdn.10xgenomics.com/image/upload/v1666737555/support-documents/CG000338_ChromiumNextGEM_Multiome_ATAC_GEX_User_Guide_RevF.pdf. Next single cell. Kit v3.1: https://support.10xgenomics.com/single-cell-gene-expression/library-prep/doc/user-guide-chromium-single-cell-3-reagent-kits-user-guide-v31-chemistry-dual-index). Single-cell suspensions were subject to the Next GEM Single Cell 3′ Kit v.3.1 protocol. Barcoded libraries were then pooled and sequenced on an Illumina NovaSeq 6000 system with associated flow cells.
The fragmented 100–125 ng of genome was displayed on the LE 220 instrument. The dual-indexed libraries were constructed using the KAPA Hyper library prep kit. A number of libraries were pooled by mass at an equimolar ratio. The library pools were hybridized by using a xGen reagent that spans a 39-Mb target region of the human genome. The libraries were hybridized for 16–18 h at 65 °C followed by a stringent wash to remove spuriously hybridized library fragments. Enriched library fragments were eluted and PCR cycle optimization was performed to prevent overamplification. The enriched libraries were amplified using KAPA HiFi master mix (Roche) before sequencing. The concentration of each captured library pool was determined through qPCR using a KAPA library Quantification kit according to the manufacturer’s protocol (Roche) to produce cluster counts appropriate for the Illumina NovaSeq-6000 instrument. Next, 2 × 150 paired-end reads were generated targeting 12 Gb of sequence to achieve around 100× coverage per library.
Extraction, Analysis, and Encoding of GRCh38.d1.vd1 from R and Python Data using TrimGalore and Samtools
All data analyses were conducted in R and Python environments. Details of specific functions and libraries are provided in the relevant methods sections above. The Wilcoxon rank-sum, proportion test, hypergeometric test, or Pearson correlation test were used to determineificance. Significant was the P values of 0.05. Details of statistical tests are provided in the figure legends and the relevant methods sections.
trimGalore preprocessed FASTq files using parameters, which included length 36, as well as other parameters. FASTQ files were then aligned to the GDC’s GRCh38 human reference genome (GRCh38.d1.vd1) using BWA-mem (v.0.7.17) with parameter -M and all others set to default. The SAM file was converted into a BAM file by using the Samtools (https://github.com/samtool/) view with parameters -Shb and all others set to default. The SortSam tool can be used to sort, mark, and default on others. The final BAM files were then indexed with the parameters set to default.
RNA sequencing library preparation using a Qubit 2.0 Fluorometer and an S2 Flow Cell with Ensembl VEP60 for optimized downstream filtering and variant annotation
Freshly dissected tumours were immediately placed in RNAlater (Thermo Fisher, AM7021) and stored at 4 °C. The instructions for the mini kit say to extract the RNA from it. Purified total RNA was quantified using a Qubit 2.0 fluorometer (Thermo Fisher), and integrity was assessed using a TapeStation 4200 (Agilent Technologies). Only samples with RNA integrity number equivalent (RINe) > 8.0 were used for sequencing. Sequencing library preparation was performed with a starting input of 100 ng RNA using the Illumina Stranded mRNA Prep Kit (Illumina, 20040534), according to the manufacturer’s instructions. In order to quantify the equimolarly pooled library, aQuant-it High Sensitivity Fluorometer and Tapestation were used. On a platform like Illumina’s NovaSeq6000, 50-bp reads were performed on an S2 flow cell.
Four samples were duplicated in order to determine the error rate. The minimum VAF threshold for downstream filtering was chosen so that duplication of mutation calls in technical replicates was achieved. This was found to be 0.02. Additionally, mutation calls were filtered to only include nonsense mutations with ≥100 read depth.
The FASTQ files were used to align the Genome Reference Consortium mouse genome 39. Mutation calling was performed using the ampliconseq pipeline (https://github.com/crukci-bioinformatics/ampliconseq) with VarDict as variant caller and a minimum allele fraction threshold of 0.01. Variant annotation was performed using Ensembl VEP60. The list of called mutations was filtered to remove variants that did not pass internal noise filters. Indels were removed because of the predilection of ENU to predominantly cause single-nucleotide variants61. If they were supported by at least five mutant reads and called in at least two amplicons per sample, variants were retained. The codons 73–84 and 126–139 of APC were covered by only one amplicon, and they were manually checked to see if they were called by more than one read. This only affected one sample (2122307_26).
NEB Q5 High-Fidelity DNA polymerase (M0491S), with primers hybridizing to exons 4 and 16 of Apc (for_261-AAAAATGTCCCTTCGCTCCT and rev_3149-CTGTGAGGGACTCTGCCTTC, respectively) was used for PCR amplification of cDNA template. Each sample had a five-digit barcode on the forward primer. PCR products of each minor clone sample were first analysed by gel electrophoresis to confirm expected size distribution (several bands due to alternative splicing and Cre-mediated excision of exon 15 with a mean size of 2,585 bp), purified, quantified and then pooled in an equimolar ratio. The library was built and sequenced on a SMRT Cell at the Earlham Institute.
mRNA was isolated from micro-dissected minor tumour clones as described above. A NEB Protoscript II First strand cDNA synthesis kit was used according to the manufacturer’s instructions. Both oligo-dT or a gene specific reverse primer (rev_3699 GCCTTTTGGCATTAGATGGA) were used.
cDNA was synthesized from 1 μg RNA using the iScript cDNA synthesis kit (Bio-Rad, 1708891). According to the instructions on a QuantStudio6 (Applied Biosystems), a Taqman Gene Expression Assay was used to perform Real-Time QuantitativePCR for Notum. The 2(2-rmDelta Delta C_rmt) method was used to determine relative fold change. All ΔΔCt values were normalized to the housekeeping genes Gapdh (Mm99999915_g1) and Rpl37 (Mm00782745_s1).
Skoufou-Papoutsaki et al. 73 described a knockout of APC using a CRISPR-based method. In brief, mouse small intestinal organoids were single-cell dissociated at 37 °C in TrypLE Express (Gibco, 12605010) and Y-27632 (1:1,000, Tocris, 1254) for 30 min. One hundred thousand cells were then put into a cell line which included a single guideRNA complex. sgRNAs were designed using Benchling (https://www.benchling.com/) and Indelphi (https://indelphi.giffordlab.mit.edu/)74. Guides were designed to lead out of frame indels and therefore, truncations at codons S96 and T619. The sgRNA was pre-Armadillo, CCTTCGCTACGGAAGTC; TGTCTGCCGGTAGA; and MCRS. The cells were then transferred into a 16-well nucleovette strip and kept at room temperature for 10 minutes. Electroporation was performed on an Amaxa 4D Nucleofector (Lonza) using the DS138 programme. After 10 min at 37 °C, cells were transferred to a 0.5 ml tube, suspended in 20 μl Cultrex and plated as described above. Once organoids were formed (usually about 7–10 days after plating), single organoids were picked using a EZGrip micropipette (CooperSurgical Fertility Solutions) under microscopic visualization. Single-picked organoids were then placed in 5 μl TrypLE Express for 10 min at room temperature, mixed with 15 μl Cultrex and replated to generate clonal organoids. The kit was used according to the manufacturer’s instructions. PCR was performed using custom designed primers and the product Sanger sequenced. Pre_Armadillo forward, GGCAGATGGGTTCAAAGGGGTAGAG was used in the primer. Pre_Armadillo reverse, AAACTCCCACGCACACACAGTACTT; There are two arms, one arm forward, one arm reverse, and one MCR reverse. The knockout score and clonality were determined using the ICE Synthego platform.
There were two types of colonoids, a primary and a metastatic one. Cells were resuspended at 2,000 cells per 40 l of Matrigel after being processed at 600g. After Matrigel domes solidified at 37 C, the wells were added with the HISC medium. Organoids were established after three passages. For non-tumour organoid culture, HISC medium was supplemented with human R-spondin 1 (1 μg ml−1; Peprotech) and NGS-WNT (0.5 M, ImmunePrecise N000). The medium was changed every 3–4 days. Organoid lines were expanded and stock vials were frozen.
The standard growth medium had advanced DMEM/F12 with 10 mM penicillin-streptomycin. 10 mM HePES, B 27 supplement, N-2 supplement, and L-glutamine are included in the list. Dissociation medium was made with 2.5% FBS and a 10 mM. penicillin-streptomycin,75 U ml1 and 50 gml1 liberase TL, are part of the penicillin family. There is a stem cell Tech called DNAse I.
Sequencing and Quantification of GRCm39 Ensembl Release 103 RNA-sequencing Experiments in Mouse using MmCMS, SPAM and FastQC
The quality of the sequence read was assessed using the FastQC tool. Adapter content was trimmed from the reads using Trimmomatic (v0.39)62. Trimmed reads were aligned to GRCm39 Ensembl release 103 for quality control purposes using STAR version 2.7.7a63 and quality control of the aligned reads was carried out using Picard tools (v2.27.3). Gene expression quantification was carried out using Salmon (v1.9.0) against indexes generated from Gencode Mouse release M30. Differential gene expression was performed using the DESeq2 package64. Genes were differentially expressed at the P value of 0.05. The Geneset enrichment analysis was done in R with the help of the clusterProfiler package. Signature scores were based on the Hallmark Pathways66 and published gene sets for mouse ISCs by Muñoz et al.67, Merloz-Suarez et al.68 and mouse small intestinal and colonic secretory signatures from Tomic et al.69. The published Wnt pathway and Apc knockout genes were included in the use70. Mouse intestinal cell-type signatures were derived from a compendium of single-cell RNA-sequencing experiments hosted at PanglaoDB71. Consensus Molecular Subtyping was performed on DESeq2 vst normalized counts using gene set collection C in the MmCMS package24 (https://github.com/MolecularPathologyLab/MmCMS). The default prediction probability is recommended by the Malla et al.72 package on thegithub.com/sidmall/PDSclassifier.
The targeted amplicon library was prepared according to the Standard BioTools protocol using the 8.8.6 integrated fluidic chip (IFC) and the Juno system. The highly interrogative of 48 samples against 8 independent panels of primers resulted in the generation of 286 amplicons for each sample. In order to quantify the amplicons from each IFC, they had to pool equimolarly. Sequencing was performed as paired end 150-bp reads on the Illumina platform.
Standard BioTools’ D3 Assay Design software was used to design a targeted panel of primers covering ten genes (Apc, Ctnnb1, Kras, Nras, Hras, Braf, Pten, Fbxw7, Smad4 and Trp53). There was an exome panel that covered 100% of the exonic regions of APCS, Ctfnb1, Kras and Trp53, but only a small amount of the other genes. All the targeted nucleotides in the panel were covered by at least two amplicons apart from Apc and Pten, which had 99.2% and 77% dual coverage, respectively.
DNA extraction from bulk or micro-dissected tumours was performed using a QIAmp DNA FFPE Tissue kit (Qiagen, 56404) according to the manufacturer’s instructions, apart from a longer lysis incubation time of 12 h at 56 °C and omission of the 90 °C incubation step. The degraded DNA was measured with a spectrophotometer. The stored is at 20 C.
The irritative segments were washed with PBS and pinned onto black pads. Tumours were visualized with a microscope under which they could be excised using fine scissors and pieces of the body.
Qupath is the method used to perform all histological quantification. Annotations based on Confetti status were first manually created for each heterotypic tumour using a section stained for RFP and GFP. Positive cells were identified using a positive cell detection feature with intensity thresholds of five and eight, which was used as a nuclear marker. For chromogenic or fluorescent duplex RNAscope staining, immunofluorescent detection of LYZ1 and UEA1, results were reported as the number of positive cells per unit area of the annotation. Ki67 staining results were reported as percentage of DAPI-positive cells.
Simultaneous detection of Lgr5 and Anxa1 and detection of Notum were performed on paraffin embedded sections using Advanced Cell Diagnostics (ACD) RNAscope 2.5 LS Duplex Reagent Kit (322440), RNAscope 2.5 LS Probe- Mm- Anxa1 (509298), RNAscope 2.5 LS Probe-Mm-Lgr5-C2 (312178-C2), and RNAscope 2.5 LS Probe-Mm-Notum-C1 (428988-C1) (ACD). Three-micrometre-thick sections were baked for 1 h at 60 °C before loading onto a Bond RX instrument (Leica Biosystems). Slides were deparaffinized and rehydrated on board before pre-treatments using Epitope Retrieval Solution 2 (AR9640, Leica Biosystems) at 95 °C for 15 min, and ACD Enzyme from the Duplex Reagent kit at 40 °C for 15 min. Probe hybridization and signal amplification were performed according to manufacturer’s instructions. Fast red detection of C2 was performed on the Bond Rx using the Bond Polymer Refine Red Detection Kit (Leica Biosystems, DS9390) according to ACD protocol. Slides were then removed from the Bond Rx and detection of the C1 signal was performed using the RNAscope 2.5 LS Green Accessory Pack (ACD, 322550) according to kit instructions. Slides were heated at 60 C and mounted using Vecta Mount Permanent Mounting Medium. The slides were imaged on the aperio AT2 so they could be seen in whole. Images were captured at 40× magnification, with a resolution of 0.25 μm per pixel.
The images were taken with a confocal microscope with a 10 objective, a 1.4– 1.7 optical zoom and 8–12 m z- steps throughout the whole thickness of the tissue. The image analysis was done using a software. All identified tumours had their Confetti status manually assessed at all of the acquired z positions. A tumour was only identified as heterotypic if it showed evidence of glands of at least two Confetti colours or one Confetti colour in the presence of unlabelled glands. The purpose of this wasn’t single inter mixed glands but rather determining their status as entrapped normal crypts.
The introduction of tumours suppressor and oncogene fields was triggered by a single injection of 4mcg of the drug tamoxifen dissolved in wine and oil. The chemical mutagenesis was performed after 10 days following field induction when 200MG ENU dissolved in the buffer.
Male and female mice of at least 8 weeks of age were used for the experiments. The Federation of European laboratory animal science Associations recommend that health monitoring be done on mice housed in a specific pathogen-free facility. Food and water were provided. Prior to the study, there had been no procedures done on the mice. When it came to survival curve generation, the mice were old before they showed signs of tumours burden. No mice were able to reach these endpoints. Randomization or blinding was not used. The results of preliminary experiments were used to make the sample sizes. All animal experiments were performed in accordance with the guidelines of the UK Home Office under the authority of a Home Office project licence (PD5F099BE) approved by the Animal Welfare and Ethical Review Body at the CRUK Cambridge Institute, University of Cambridge.
Source: Polyclonality overcomes fitness barriers in Apc-driven tumorigenesis
Real-time PCR genotype of gastrointestinal epithelium-specific inducible Cre and R26R-Confetti53 alleles
The intestinal epithelium-specific inducible Cre (Villin-creERT2)51 (JAX020282) line was crossed with Apcfl/+ (ref. 52) and R26R-Confetti53 (JAX017492) lines on a C57BL/6 background to obtain mice heterozygous for these alleles. Additionally, the LSL-KrasG12D54 (JAX008179) and Trp53fl/fl55 alleles were used in some experiments. Transnetyx used real-time PCR to perform genotyping.
To perform the Confetti labelling simulation (Extended Data Fig. 1f), a custom PERL script was written to randomly assign a Confetti label to crypts in a 10 × 100 field of unmarked crypts, using the observed Confetti fluorophore frequencies (CFP 2.7%, YFP 4.1%, and RFP 3.8%). The number of patches (contiguous crypts with the same fluorophore) was quantified for each simulation (a total of 1,000 simulations were run for each fluorophore), and the distribution of coloured crypts within particular patch sizes was calculated.
Additionally, a simulation making use of the observed growth rates of tumours in the Apchet + ENU model was created. For each confocally imaged region of intestine, a number of points were initialized at t = 0 after ENU with each point attributed a Confetti label (based on observed frequencies of CFP, RFP, YFP and uncoloured crypts) and a growth rate sampled from the distribution of observed growth rates. The simulation was halted at the humane endpoint reached by the mouse. For each simulation there was a tally of the number of crashes that resulted in tumours. The simulations were done for a long time. The observed number of tumours was compared to the expected number by using a pair of t-tests.
To investigate whether heterotypic tumours arise in regions of highest density, the spatstat package was used to calculate local spatial tumour density within each imaged bowel segment. The local spatial density was extracted at the location of each tumour, and densities at heterotypic tumours were compared to those of non-heterotypic tumours using a Q–Q plot and the Kolmogorov–Smirnov test. The hypothesis is that the density distributions would be higher if clustering occurred in high density regions.
To quantify the growth dynamics of heterotypic and homotypic tumours (Fig. 4d), a mixed-effects model was built using the package nlme using mouse identity as a random effects term. The rate of tumour expansion was quantified during the exponential growth phase (that is, between timepoints 40 and 63 days after ENU).
Visualization and statistical analysis of data were performed in the R statistical computing environment (version 4.2.3) or GraphPad Prism version 10.2.2 (341). The Benjamini–Hochberg method was used for multiple testing correction of P values. All immunostaining and RNAscope experiments were performed on at least three independent biological replicates (three different mice). Micrographs depict representative data derived from at least three independent biological replicates. The figures and legends show the corresponding P values. Box plots display the distribution of data using the following components: lower whisker show the smallest observation greater than or equal to lower hinge minus 1.5× IQR; lower hinge shows the 25% quantile; the centre line shows the median, 50% quantile; the upper hinge shows the 75% quantile; the upper whisker shows the largest observation less than or equal to upper hinge plus 1.5× IQR.
We applied MAGIC (v.3.0.0) imputation59 to normalized, log-transformed count matrices to denoise and recover missing transcript counts due to dropout. Imputation was performed using conservative parameters. In order to analyse gene correlations in cancer patients, Imputed values have to be used, as well as visualization of gene expression or gene signature expression.
Patients that had signed pre-procedure informed consent for the biospecimen collection and were identified through a chart review were selected for the study. No statistical method was used to determine the sample size. Freshly-resected surgical tissue was processed into single-cell suspensions for scRNA-seq analysis and organoids, where enough tissue was available, to generate organoids. The portions that were fixed in formalin and embedded in paraffin, were also fixed in formalin. Tissue was generally processed within 1 h of surgical resection. Archival formalin-fixed, paraffin-embedded (FFPE) clinical tissue blocks for immunostaining were identified by database search and chart review. An expert gastrointestinal pathologist (J.S.) oversaw the processing and interpretation of histologic data. The patient was tracked longitudinally through their clinical course and the tumour tissue surplus from any subsequent procedures was collected.
Clinical data, including baseline demographic data and previous treatments (Supplementary Table 1 and Supplementary Fig. 1), were abstracted through manual review of patient electronic medical records by board certified medical oncologists (M.L. and K.G.), collected as part of institutional review board approved protocols (MSK IRB, 14-244 and 22-404). The time to each treatment event was calculated from the date of diagnosis for each patient. Study data were collected and managed using REDCap electronic data capture tools hosted at MSKCC on secure central servers. 17 out of 31 patients had multiple metastatic sites at the time of surgery, and had >50% of tumour sites remaining after surgery. 17 out of 31 patients had early-onset CRC (age of diagnosis, <50 years). From 27 patients, 27 of which were identified as having expected mutations53, the MSK-IMPACT targeted exon sequencing was performed. 1b). Consistent with the low percentage (<5%) of metastatic CRC that is mismatch repair deficient/microsatellite instability high, only one patient in our cohort had an microsatellite instability indeterminate tumour. The collection of clinical data was stopped.
We collected 50–300 mg of freshly resected surgical tissue in 5 ml of IGFF organoid medium (Advanced DMEM/F12 (AdDF12; Thermo Fisher Scientific), GlutaMAX (2 mM, Thermo Fisher Scientific), HEPES (10 mM, Thermo Fisher Scientific), N-acetyl-l-cysteine (1 mM, Sigma-Aldrich), B27 supplement with vitamin A (Thermo Fisher Scientific)) supplemented with primocin (100 μg ml−1, InvivoGen), plasmocin (50 μg ml−1, InvivoGen), penicillin–streptomycin (100 μg ml−1, Thermo Fisher Scientific), Amphotericin B (2.5 μg ml−1, Cytiva), nystatin (250 U ml−1, Millipore Sigma). For primary tumours, you would put the specimen in a 15 cm petri dish, wash it three times with DPBS, and minimally chop the Tumours using sharp sterile blades.
Non-tumour tissue was transferred into a 50 ml tube pre-filled with 25 ml dissociation/chelation buffer (8 mM EDTA, 0.5 mM DTT, DNase I (100 U ml−1, Millipore Sigma)). A maximum of 30 min was allowed when the mucosal fragments were kept in rotation at 4 C. The tissue fragments were assessed every 10 min under an inverted microscope. Dissociation was stopped before 30 min if at least 30% of the material appeared to be broken into smaller groups. Next, the crypt solution was filtered through a 1 mm cell strainer (PluriSelect) to separate individual crypts or small crypt clusters from large chunks of undissociated mucosa. Dissociation was quenched with an equal volume of DPBS. At this point, the 1 mm filter was flipped and inverted into a fresh 50 ml tube. Up to 25 ml of DPBS supplemented with antibiotics was flashed through the inverted filter to recover the undissociated mucosal tissue. After manually shaking the suspension of mucosal tissue fragments (approximately 5 times), the collection of clusters of colonic crypts was reattempted as described above. Up to three more fractions of crypt suspensions were collected from the iteration of both manual and filtration steps. The Crypt suspensions were washed three times, with each centrifugation step carrying 100g at room temperature. Based on visual inspection under an inverted microscope, one or more crypt suspensions were selected for subsequent processing according to the size and integrity of the crypts, and either processed separately or pooled together if individual suspensions were assessed to have low crypt content.
If there were blood traces under an inverted microscope, the cell pellet was resuspended in a 1-8 m ACK lysis buffer. Quenching was performed with three volumes of DPBS supplemented with antibiotics, followed by an additional wash to remove ACK traces. Organoid generation or both was then processed for the resulting cell pellet. Tissue processing procedures were extensively and iteratively changed to maximize the retrieval of high quality single-cell suspensions for downstream analyses.
For validation, organoids underwent targeted exome sequencing by MSK-IMPACT53 and key oncogenic genomic alterations were identified by OncoKB55 (see below). Diagnostic tissue from originating tumours was sequenced to confirm that these alterations were conserved in each derived organoid line. Organoids were verified on the basis of short tandem repeats at the time of establishment and before every experiment, and were routinely tested for mycoplasma contamination (MycoALERT PLUS detection kit, Lonza).
We used fetal gut cell atlas data from aborted human embryos to capture the development of human intestinal cells from a fetal progenitor state to differentiated crypts. We downloaded a raw H5AD file from the authors containing all epithelial cells from fetal donors and limited our analysis to 8,408 cells originating from the large intestine of first and second trimester samples.
Raw count matrices were normalized to the median library size and log-transformed with a base of e and pseudocount of 0.1. We then selected highly variable genes (HVGs) using the highly_variable_genes function in Scanpy (v.1.9.1) and flavour = seurat_v3 (we chose bins = 40). We kept the top 50 genes within each bin, for a total of 2,000 HVGs. Moreover, for all datasets (besides the fetal colon dataset), we included genes with known relevance to normal colonic cell types (41 genes) and cell states associated with inflammatory disease, injury of the colon, and regulation of REST and EMT (56 genes) (a complete list of genes is provided in Supplementary Table 7). We will add 2,094 genes, including both HVGs and manual additions as HVGs. We performed PCA using only HVGs, while retaining the number of principal components that explain 75% of the variance. For all datasets as well, we chose a number of PCs explaining 75% of variance.
For all two-dimensional embeddings, we used the Scanpy (v.1.9.1) neighbours function to compute a k-nearest-neighbour graph on the PCs based on Euclidean distance and k = 30. To visualize the global CRC cell atlas (Extended Data Fig. 2b,e,h,i), non-tumour epithelium (Extended Data Fig. 2f) and human fetal gut cell atlas (Fig. 2c), we generated projections using the UMAP implementation in Scanpy (v.1.9.1), with min_dist = 0.3–0.5 and init_pos = paga. The data is available to show subsets of the cells, including all treated cells. All the tumour cells are included in the extended data fig. 5e–j) and patient KG146 cells (Fig. 2a and Extended Data Fig. 9b,c), we used force-directed layouts, which provide a more intuitive representation of cell state transitions and the local relationships between subpopulations, using Scanpy (v.1.9.1) with the ForceAtlas2 layout and init_pos = paga. The Python package matplotlib (v.3.6.0) was used to produce all plots.
The protocols used in all animal experiments were approved by the Iacac. NSG (NOD.Cg-PrkdcscidIl2rgtm1Wjl/SzJ, 005557) mice were obtained from the Jackson Laboratory and were transplanted at 6 weeks of age86. A specific-pathogen-free facility, with a 12 h–dark cycle under temperature and humidity, and ad libitum access to traditional diet, or a combination of the two, was used for maintenance of the mice. For orthotopic caecal and intrahepatic injections, OKG146P, OKG146Li, OKG136P or OKG136Li organoids were transduced with pLenti-PGK-Akaluc (AkaLuc) or pLenti-PGK-tdTomato-Akaluc (TdT-AkaLuc) virus (subcloned from pLenti-PGK-Venus-Akaluc(neo)) and HR180-LGR5-iCT plasmids through Gibson assembly (Addgene, 124701, 129094). The lines expressing shRNAs to PROX1 or Renilla control were transduced with pLenti– Akaluc. For caecal injections, 200,000 cells from each organoid line were mixed with 50% Matrigel in 10 μl of HISC and injected into the caecal submucosa of NSG mice. For hepatic injections, 500,000 cells from each organoid line were mixed with 50% Matrigel in 10 μl of HISC and injected under the liver capsule of NSG mice. Weekly bioluminescence imaging was performed on the IVIS Spectrum Xenogen instrument (Caliper Life Sciences) and analysed using Living Image software v.2.50. Experimental group sizes were practically determined on the basis of five mice per cage, with n ≥ 5 age- and sex-matched mice per group. Animals were assigned groups based on relevance. When the tumour size exceeded 10% of body mass, and when the animals showed signs of respiratory distress or illness, such as hunched posture, failure to groom or less than 10% weight loss, the experimental end point was reached. None of the experiments exceeded these limits. At the humane end point, the animals were euthanized and tissues were collected. Where needed, tissues were fixed with 4% paraformaldehyde. The investigators were blinded to the mouse group.
Organoids from Matrigel were recovered using 3 mM. The lysed EDTA was washed with dPBS and then lysed with a 1Ripa buffer supplemented withPPI and benzonase. The protein concentration was determined using Pierce BCA assay (Thermo Fisher Scientific, 23227). The 10 g per sample was separated by the use of a technique calledSDS–PAGE, which uses a reagent called Bi-Tris polyacrylamide. The following antibody were added to the membranes: mouse anti- -actin (1,1,000,Thermo Fisher Scientific, AM4302), and rabbit anti- PROX1, followed by secondary antibody incubation with 488 anti. Western blots were quantified using ImageJ (v.1.53t)84.
In order to perform downstream analyses, we only retained cells from the areas that were demarcated based on histology. We included this preliminary filtering step to (1) remove most stromal cells, therefore reducing the computational cost downstream; and (2) distinguish tumour cells from normal epithelium using histological evidence, as this was not possible from marker expression alone (for example, due to expression of stromal marker genes in basal-like tumour cells). As we use only COMET data to compare CDX2+ intestinal and PROX1+ fetal-like cells, we first restricted our analysis to cells expressing either PROX1 or CDX2 above a stringent level of background (99th percentile of expression in unmasked regions calculated separately for each image). We then removed 6 samples with fewer than 1% PROX1-expressing cells, leaving 7 samples. We labelled each cell if it was expression of one or both genes above the mean.
An experienced physician manually annotated 167 FOVs to indicate if they have a high background signal in response to high levels of CK5 staining. To identify high-background images in the remaining 435 FOVs, we first found the highest level of background CK5 signal (10th percentile of expression across all cells in the FOV) within FOVs labelled low-background (around 0.0068). All the unlabelled FOVs were classified as high background. 327 unlabelled FOVs with low background were used in subsequent analyses, and 108 with high background were removed. In total, we used 456 FOVs with a low level of CK5 background expression in all figures involving Vectra panel 2.
The marker expression levels are determined by summing the brightness values within the cell boundaries. To make sure that our analysis was not influenced by cell size, we took the per-cell expression for each channel and divided it by the cell’s boundary size. Once normalized, all cells were pooled into cell-by-expression matrices within the same imaging technology and panel (Vectra panel 1, Vectra panel 2 and COMET) for downstream analyses and annotated with patient- and sample-level metadata.
We ran Mesmer (v.0.12) on these images with the default parameters to predict cell boundaries, and calculated the cell size, eccentricity and centroid of each cell boundary using the regionprops function (default parameters) in the Python skimage (v.0.23.2) package. When running Mesmer (v.0.12), we first subsampled COMET images by half so that they would fit in system memory (128 GB of memory). For both cell size and DAPI expression, we found the distributions of segmented cells produced by Mesmer (v.0.12) were bimodal and the lower mode contained primarily empty regions and not real cells. We therefore filtered out all predicted cell boundaries below threshold values of 30 px2 cell size (estimated from the distribution) and a log2-normalized DAPI intensity of 11 (COMET), 1 (Vectra panel 1) and 1 (Vectra panel 2) (estimated from the distribution). This resulted in a COMET dataset of over six million cells, a Vectra panel 1 dataset of over five million cells, and a Vectra panel 2 dataset of over five million cells.
In order to summarize our classification, we had to use coarser cell typing. Thus, to summarize our organoid sample classifications, we aggregated the probabilities of different cell states into three generalized categories; ISC-like, combining TA/Proliferative for ISC/TA, absorptive-like and secretory-like for differentiated intestine, and combining fetal/injury repair, neuroendocrine and squamous for non-canonical. These groupings can be used to understand the likelihood of a cell being from any of the states that were combined. The probabilities are plotted using the python-ternary package.
The expression trends for the transcription factors and the fetal progenitor signature score are computed by using Palantir to calculate the delineations of the canonical to non-canonical tumours axes. As we are interested in transcription factors that drive the transition from canonical to fetal cell states, we focused on those with peak expression just prior to entering non-canonical states along the DC of each patient. We identified the first maxima of the trend in the fetal progenitor signature on the DCs of patients KG146,KG182,KG151 andKG183. 7b). When the derivative first shifts from positive to negative, Maxima is the first inflection point of the trend. Trends for KG150 and KG183 lack a first-derivative inflection point, so we used the position of the maximum value for these patients. We then calculated the Pearson correlation between the expression of each transcription factor and the fetal progenitor gene signature score using only cells at positions along a patient’s DC which precede the signature score peak. The correlation values for each transcription factor were determined by this. We focused only on transcription factors with a minimum correlation of r = 0.5 in patient KG146 (leaving 14 transcription factors) and r = 0.2 in all four patients (leaving 5 transcription factors).
For the remaining six transcription factors, we determined their treatment response in HISC-grown organoids by computing the log-transformed fold change between irinotecan-treated and untreated conditions. log-transformed fold changes were calculated from single-cell data only using cells classified as non-canonical (see the ‘Mapping organoid data to patient tumour’ section) (Extended Data Fig. 11b).
Building bridge between in vitro and in vivo multispectral imaging systems using the Vectra Multispectral Imaging System (Akoya)
Seven-colour multiplex-stained slides were imaged using the Vectra Multispectral Imaging System version 3 (Akoya). Scanning was performed at ×20 (×200 final magnification). The Cy5 is one of the filter cubes used for multispectral analysis. Vectra image analysis software was used to create a library of the fluorescent peaks in this study. Using multispectral images from single-stained slides for each marker, the spectral library was used to separate each multispectral cube into individual components (spectral unmixing) allowing for identification of the seven marker channels of interest using Inform v.2.4 image analysis software.
Finally, we supplied the augmented affinity matrix from step 2 to the PhenoGraph (v.1.5.7) classify function11 with the default parameters. This function calculates the probability of random walks from unlabelled cells to a class of labelled cells in a sample and also converts the affinity matrix into a row normalized Markov matrix. Each unlabelled cell is given a cell-state label with a maximum probability.
Due to the differences between in vivo and intericulum data, our approach consisted of a joint PCA and UMAP, which caused label transfer between similar cells to be useless. We follow the approach outlined previously 45 to bridge between datasets. First, we computed the nearest neighbor graph in the separate dataset, followed by mutual nearest neighbours between the samples using the Harmony77. Importantly, we used the cosine metric to quantify the distance between cells across samples, as this metric is less sensitive to technical artifacts and better reflects conserved biological states in both in vivo and in vitro samples78. The number of mutual neighbours is higher due to the strength of the MNN graph.
The Scanpy dotplot function is used to plot cells with the top-notch expression for a module. 5b,c). To assign cells as top-scoring for a Hotspot module, we z-normalized all Hotspot module scores across cells, and then required a top-scoring cell to very specifically express a given Hotspot module and not express any others. To achieve this, we had to have a score above the mean for all other modules. The percentile scores used for other sections when identifying cells that are high for a module were found to be more narrow than the criteria we used.
The module trends were visualized using generalized models to fit gene expression along with the Palantir-computed pseudotime. The s.d of each expression bin was represented by the residuals of the fit.
There are four patients with tumours that have the most cells with non-canonical fates. We used Palantir (v.1.2)42 to investigate these two fates and the genes underlying their respective fate transitions. As an input, Palantir requires an initial state, and as the output, it computes terminal fates and provides a cell-fate map that assigns a probability for each cell to differentiate into each terminal fate. Palantir also outputs a pseudotime alignment of cells from the initial to each of the terminal states and, therefore, by combining pseudotime and fate probability for each cell, it can provide branching gene trends leading to each terminal fate (by weighing the contribution of each cell to the gene trend based on the fate probability). There was insufficient number of non-canonical cells in the patient that led to Palantir being run separately. We selected cells with the highest imputed expression of LGR5 as the initial state, motivated by their identification as cells of origin in CRC studies. Notably, Palantir has been shown to be robust to the exact choice of starting cell. Running a number of DCs and waypoints with an eigengap gave a different branching trajectory from the state to two CDX2 terminal cells. We disregarded three additional branches in KG146 and KG150 that probably represent canonical-state trajectories, as the terminal cells express CDX2 and differentiated intestine markers including FABP1 and TFF3.
For all of the samples in the LARC and TCGA-COAD cohorts, we calculated ssGSEA enrichment scores as described in the ‘ssGSEA analysis’ section using our fetal gene signature as input. For each cohort, we then collected (1) highly enriched samples with ssGSEA enrichment scores >1 s.d. above the mean enrichment score among all samples; and (2) lowly enriched samples with enrichment scores <1 s.d. below the mean. We used the Python lifelines package to perform a log-rank test between the groups. 7g,h).
The fetal signature we used was compared to previously published signatures. For each pair of signatures, we calculated the Jaccard index (number of genes shared between signatures divided by total number of genes in both signatures), demonstrating that existing signatures are clearly distinct from our fetal signature and lack consensus (Extended Data Fig. 7b). The amount of core fetal signature genes in each dedifferentiation signature is normalized to the total number of genes in that signature.
Stem cells make up 85% of all cells, and progenitor cells make up 42% of the samples. By contrast, second-trimester samples consist of mature colon mucosal cell types exclusively, and exhibit strong expression of LGR5, TFF3, SLC26A3, NEUROD1 and POU2F3 (corresponding to ISCs, goblet cells, mature enterocytes, enteroendocrine and tuft cells, respectively). We therefore concluded that the separation between the first- and second-trimester samples captures the distinction between progenitor-like cell types and colonic crypts.
Source: Progressive plasticity during colorectal cancer metastasis
Entropy analysis of Hotspot modules for the tumour TCGA-COAD study31 Using GAMs and Smoothing Functions
The module score trends along the DC axes were analysed by using GAMs and smoothing functions from Palantir (v.1.2)42. 7a). GAMs increase robustness and reduce sensitivity to density differences, and cubic splines are effective in capturing non-linear relationships. We fitted trends for a module score using a regression model on the DC values (x axis) and module score values (y axis or colour intensity). The smoothed trend was created by dividing the data into 500 equally sized bins and using a regression fit to predict module scores at each bin. The module score trends are visualized from the 20th percentile to the highest saturation. 7b).
We used entropy to evaluate the patient-specificity of each Hotspot module using the following procedure: (1) sample 357 cells per patient with replacement in our tumour dataset to ensure even cell distribution across patients. (2) For each module, determine the subset of high-scoring cells with module scores greater than 1 s.d. above the mean. The Shannon entropy of patient labels can be computed using the scipy.stats.entropy function. To calculate the Shannon entropy, we first built a k-NN graph with k = 60 on the multiscale space embedding of all epithelial cells; multiscale space was computed on the top 19 DCs (chosen by the knee-point of DC eigenvalues) using Palantir (v.1.2). The first steps are repeated 100 times before the plot of the distributions is visualized using the function in Python Seaborn.
For the purpose of comparing the scores for each module between the two groups, we separated the enrichment scores into two groups based on the status of the patient.
For TCGA, we downloaded and analysed RNA-seq data for 445 tumour samples from the TCGA-COAD study31. RNA raw counts were retrieved using TCGAbiolinks (v.2.26.0)71 and genes with a count of 0 across all the samples were removed, as well as genes that had multiple associated gene symbols or no gene symbol. The VST transformation was performed using the DESeq2 (v.1.38.3) package72. The ssGSEA analysis was done using the R package GSVA. In the cohort, 8.6% of patients have a survival status of living and an OS follow-up time of 6 months, while 4.4% have no new tumours.
The log-ratio of labelled cell fractions was visualized as the non-canonical module prevalence. The Hotspot module grouping and Annotation section describes the non-canonical module classifications. Although cells can express a variety of non-canonical modules, we found that they do not express both of the two very highly.
There were 2,003 genes retained for calculating local correlations and downstream clustering. We used the create_modules function in Hotspot (default parameters except for minimum_gene_threshold = 20 and core only = False) to obtain a preliminary set of 37 co-varying gene modules (gene assignments to modules are shown in Supplementary Table 4).
Source: Progressive plasticity during colorectal cancer metastasis
A Scanpy Analysis of the Cancer Cells in Insular Colon Samples. II. Biological Interpretation of Canonical Modules
Canonical: modules describing canonical intestinal cell types and processes such as epithelial differentiation, mucus production and small-molecule transport, which are critical for maintaining normal intestinal function.
We focused on 23 of the 37 modules, and did not explore a single module annotated as cell cycle/ proliferation, cell stress, or cilia. We manually grouped 19 module into 6 groups after arriving at the same biological interpretation for all modules in the group, and then ensuring the local correlations between genes of grouped modules were high on average. 5a,b. There were six groups and four single modules that resulted in ten final genes. 5a,b and Supplementary Table 4).
We performed differential expression analysis of the cells against each other, using the normalized, log-transformed count matrix for the non-tumour cells. The final ISC gene signature consisted of the top 100 ranked DEGs (P < 0.01 for all genes included; Supplementary Table 2). We calculated a gene signature score on the z-normalized expression of these genes using the score_genes function in Scanpy (v.1.9.1) (Fig. 3c).
We identified the cancer cells. 3c–e) using the following criteria: (1) evidence of copy-number alterations (CNAs) compared with cells originating from non-tumour colon samples; and (2) clustering that is distinct from non-tumour epithelial cells.
We partitioned the clusters based on the marker genes that were expressed. 3a,b We used the Scanpy function to score the expression of genes from the ref. 61, similar to the strategy used in that study (signatures for each compartment are shown in Supplementary Table 7). Each cluster was assigned to a particular compartment.
To generate all gene signature scores in our study, we used the Scanpy (v.1.9.1) score_genes function subtracts the expression value of genes of interest from their expression value in a set of reference genes. To account for expression-level differences across genes within signatures, we provided z-normalized expression data as the input for this function.