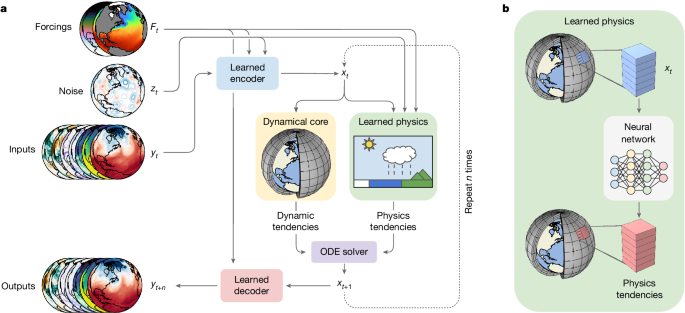
Predicting long-term climate trends and weather is easy with the help of the artificial intelligence of Google
A Supercomputer Approach to Climate Modeling: From ECMWF-ENS and NeuralGCM to GraphCast and ERA5
Ensembles are essential for capturing intrinsic uncertainty of weather forecasts, especially at longer lead times. Beyond about 7 days, the ensemble means of ECMWF-ENS and NeuralGCM-ENS forecasts have considerably lower RMSE than the deterministic models, indicating that these models better capture the average of possible weather. There is a proper scoring rule that is sensitive to full marginal probability distributions that is called the CRPS. The model we run has lower error compared to the model we use, which is from ECMWF-ENS. 2a,c,e and Supplementary Information section H), with similar spatial patterns of skill (Fig. 2b,f). A necessary condition for accurate forecasts is a spread-skill ratio of less than one.
We use the ENS model as a reference baseline to see which model will achieve the highest performance in most lead times. We assess accuracy using (1) root-mean-squared error (RMSE), (2) root-mean-squared bias (RMSB), (3) continuous ranked probability score (CRPS) and (4) spread-skill ratio, with the results shown in Fig. 2. We provide scorecards, metrics for additional variables and levels and maps in the Supplementary Information section.
We examined how much NeuralGCM, GraphCast and ECMWF-HRES capture the wind balance in mid-latitudes. A recent study16 highlighted that Pangu misrepresents the vertical structure of the geostrophic and ageostrophic winds and noted a deterioration at longer lead times. GraphCast shows an error that gets worse with lead time. In contrast, NeuralGCM more accurately depicts the vertical structure of the geostrophic and ageostrophic winds, as well as their ratio, compared with GraphCast across various rollouts, when compared against ERA5 data (Extended Data Fig. 3). However, ECMWF-HRES still shows a slightly closer alignment to ERA5 data than NeuralGCM does. In the initial days, NeuralGCM has only slightly degraded the representation of the wind’s vertical structure, and no noticeable changes thereafter.
“Traditional climate models need to be run on supercomputers. This is a model you can run in minutes,” says study co-author Stephan Hoyer, who studies deep learning at Google Research in Mountain View, California.
Scott Hosking is a researcher at the Alan Turing Institute in London and he says that the issue with pure machine-learned approaches is that they only ever training it on data that is already seen. “The climate is continuously changing, we’re going into the unknown, so our machine-learning models have to extrapolate into that unknown future. By introducing physics into the model, we can ensure that our models are physically constrained and cannot be used in a way that is unrealistic.
NeuralGCM is an area that Hoyer and his colleagues are very interested in further refining. We are modelling the Earth’s system in the atmospheric component. It is the part that affects day to day weather the most. He adds that the team wants to incorporate more aspects of Earth science into future versions, to further improve the model’s accuracy.