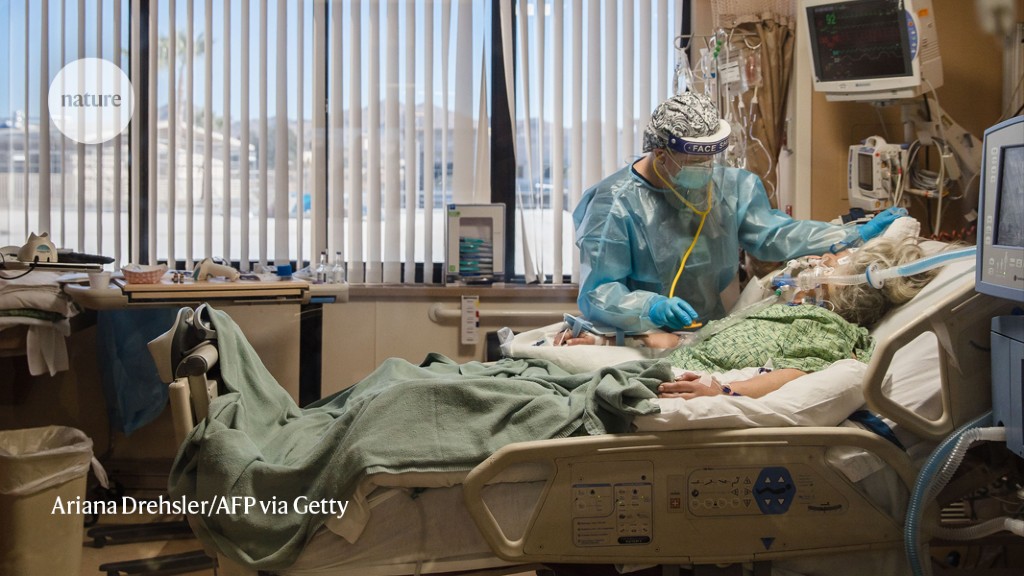
A genetics study offered clues as to why COV is life threatening for some people
Early Stages of COVID-19: The Immune System’s Role in the Development of a Treatment for Associated Severe Diseases
In the study which was published on 17 May in Nature 1, the immune system’s role in the late stages of particularly severe COVID-19 was noted. The results could one day contribute to the development of therapies for COVID-19 — and potentially other diseases that cause acute respiratory distress or sepsis.
The study’s lead author says the processes are likely to be active in other conditions. All of the work that we did will be relevant to other groups of patients that we have not identified yet.
During the first wave of the United Kingdom’savian epidemic, data was collected from people who were diagnosed with the respiratory illness. Many staff members were scared to go on the ward at that time. The 2003 coronaviruses outbreak had a mortality rate of around 10% and hit health-care workers particularly hard. “We were nervous,” says Baillie. “Certainly, in the first wave of the pandemic, and for some people in subsequent waves, it seemed like a considerable personal risk.”
“It definitely expands our understanding of the genetic determinants of severe COVID,” says Brent Richards, a human geneticist at McGill University in Montreal, Canada. An investigator is working on the COVID-19 Host Genetics Initiative, a global effort in which scientists from 54 countries share data.
The diversity of that effort will provide a crucial counterpart to the GenOMICC study, which is predominantly drawn from participants of European descent, says Richards.
In addition to the new findings, it is important that the UK study has replicated many of the findings by other groups, says Alexander Hoischen, a geneticist at Radboud University Medical Center in Nijmegen, the Netherlands. When trying to tease out the contribution of common genetic variant to disease, it’s crucial to repeat results across data sets.
This initial findings will need a lot of work to be able to turn them into new therapies. “That is still a long trajectory,” says Hoischen.
But putting together these large consortia of investigators and taking the first steps are considerable triumphs, he adds. In the early days of the H1N1 epidemic, it was unsure of how participation in large undertakings would benefit individual scientists. “Many of them acted as purely human beings,” he says, instead of worrying about their careers. “It illustrates the degree of solidarity that we really witnessed in COVID times.”
Using the Su SiE model 27, we made sets for independent signals using a method called conditional analysis. As for conditional analysis, we used a European reference panel and the meta-analysis results of the whole critical illness cohort. We performed analyses in 1 Mb windows centred on the lead variants identified through conditional analysis. In cases in which windows for multiple variants overlapped, they were joined into a single window. We used the SuSiE summary statistics model to set expected number of independent signals and number of identified signals. The models didn’t converge in 500 iteration, but have been excluded. The public disequilibrium information for non-Finish Europeans was used as a reference. Full data for all variants included in credible sets are included in Supplementary Table 5.
We performed an analysis summarizing the genetic associations at the gene level using the mBAT-combo method28. We used the COVID ‘all critical cohorts’ meta-analysis (GenOMICC, HGIv6 phenotype A2, SCOURGE and 23andMe) summary statistics. As this is a trans-ethnic meta-analysis, we used a mixed ancestry linkage disequilibrium reference panel, consisting of 3,202 1000 Genomes phase 3 samples. We considered a list of protein-coding genes with unique ensemble gene ID based on the release from GENCODE (v.40) for hg38, which can be found on the mBAT-combo website (https://yanglab.westlake.edu.cn/software/gcta/#mBAT-combo). The gene region was taken to span 50 kb upstream and 50 kb downstream.
Significant genes in the TWAS and metaTWAS were selected for a colocalization analysis using the coloc R package. The lead SNPs and a region of 200 Mb around the gene were used to colocalize with significant genes in the TWAS with eQTL summary statistics data on the region from GTExv8 lung, GTExv8 whole blood, eQTLgen or monocyte eqtl. As in our previous analysis2, we first performed a sensitivity analysis of the posterior probability of colocalization (PPH4) on the prior probability of colocalization (P12), going from P12 = 10−8 to P12 = 10−4, with the default threshold being P12 = 10−5. eQTL signal and GWAS signals were deemed to colocalize if these two criteria were met: (1) at P12 = 5 × 10−5 the probability of colocalization PPH4 > 0.5; and (2) at P12 = 10−5 The main hypothesis was the probability of independent signal. These criteria were chosen to allow eQTLs with weaker P values, owing to lack of power in GTEx v.8, to be colocalized with the signal when the main hypothesis using small priors was that there was not any signal in the eQTL data.
To detect eQTLs, untreated primary monocytes were prepared from 174 healthy individuals of Northern European (British) ancestry recruited through the Oxford Biobank. Poly(A) The Oxford Genome Centre used the illumined HiSeq-4000 machines to sequence 100 bp of RNA. Reads were aligned to CRGh38/hg38 using HISAT2 with the default parameters. High mapping quality reads were selected on the basis of MAPQ score using bamtools. Duplicate reads were marked and removed using picard (v.1.105). Samtools was used to calculate the statistics. Read count information was generated using HTSeq and normalized using DESeq2. Sample contamination and swaps were detected by comparing the imputed SNP-array genotypes with genotypes called from RNA-seq using verifyBamID. Genotyping was performed with Illumina HumanOmniExpress with coverage of 733,202 separate markers. Genotypes were tested with SHAPEIT2 before being imputed with PBWT. Poly(A) RNA was paired-end sequenced at the Oxford Genome Centre using the Illumina HiSeq-4000 machines. The Vcc tool was used on the genetic variation data to make it easier to remove indels and SNPs with a less than 0.04 rate of all genes.
GSMR5 was performed. The outcome of the colvid meta-analysis were the pQTLSs from the ref. There are 30,000 individuals of European ancestry who were randomly sampled from the UK Biobank and50,000 individuals of linkage disequilibrium to missense. GSMR was performed for all exposures for which we were able to identify two or more suitable SNPs. In order to meet the criteria, there were three factors: 1) exposure association P 5 108; 2) linkage disequilibrium clumping lead SNPs only; and 3) the deletion from HEIDI-outlier filters. The effect sizes and standard errors were estimated in the supplementary note. There is a report titled “32.” We considered as significant those exposure–outcome pairs with FDR < 0.05.
The hospitalized patients have been hospitalized with a laboratory-confirmed outbreak of the disease. GenOMICC, Brazil, GenOMICC Saudi Arabia, and a few other countries have data that has been removed from the analysis. An extended description can be found in Supplementary Table 1 and a table with the studies included can be found in Supplementary Table 14.